Watch: Drone navigates through forests and buildings in real time without intricate maps
MIT Computer Science and Artificial Intelligence Laboratory researchers devised a new system called NanoMap.
Researchers at MIT Computer Science and Artificial Intelligence Laboratory (CSAIL) have devised a way to teach small drones to see and detect the environment they fly through rather than depend on strict, intricately put together maps for navigation.
Traditional navigation systems usually have images or maps which the drone uses to stay on path constantly. While this might work in a static and ideal environment, that does not really exist in the real world.
Considering these practical challenges, the team of researchers realised using preset path or instructions at all times was futile. They then developed NanoMap — a system that positions a drone in a path where it can look and process its surroundings as it flies in real-time
Using this system, a drone can fly through a dense forest or an urban environment at a constant 20 mph speed without bumping into any obstacles. The researchers said that uncertainty in the flying environment has been coded into the AI to enable the unmanned objects fly on their own without referring to maps.
"Overly confident maps won't help you if you want drones that can operate at higher speeds in human environments," said graduate student Pete Florence, lead author on a new related paper. "An approach that is better aware of uncertainty gets us a much higher level of reliability in terms of being able to fly in close quarters and avoid obstacles."
Explaining further, the research team said in a statement that when unpredictable objects get in the way of a drone, it will not be able to adapt to the sudden changes and is likely to crash. If the drone is off target even by a small margin, quickly adapting to changes might also not be possible.
NanoMap, on the other hand, makes use of a depth-sensing system that stitches together a series of measurements based on the drone's immediate surroundings. It then creates two plans — one for its current field of view, and second prepares the drone to move around in hidden fields of view based on what it has already seen.
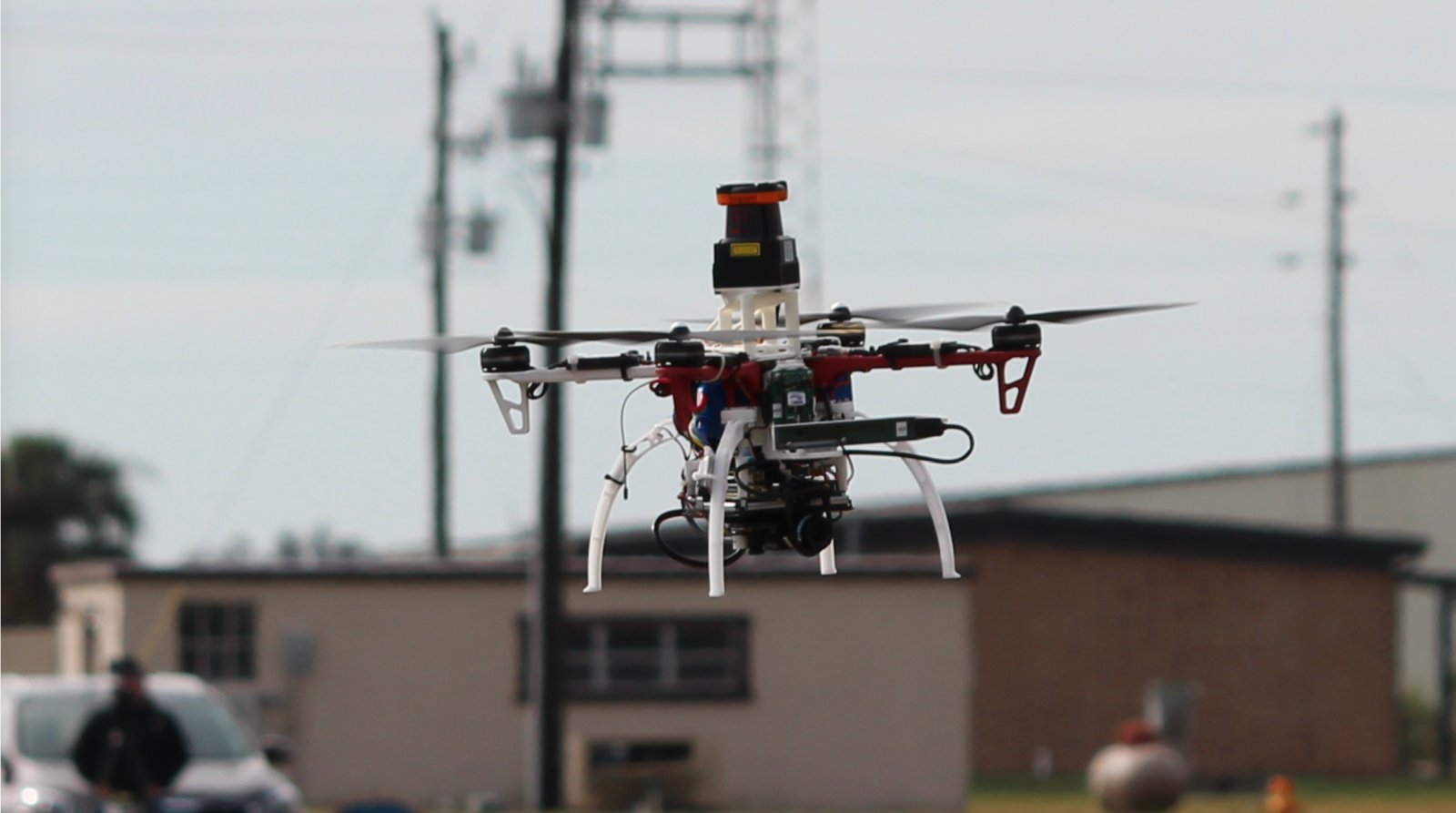
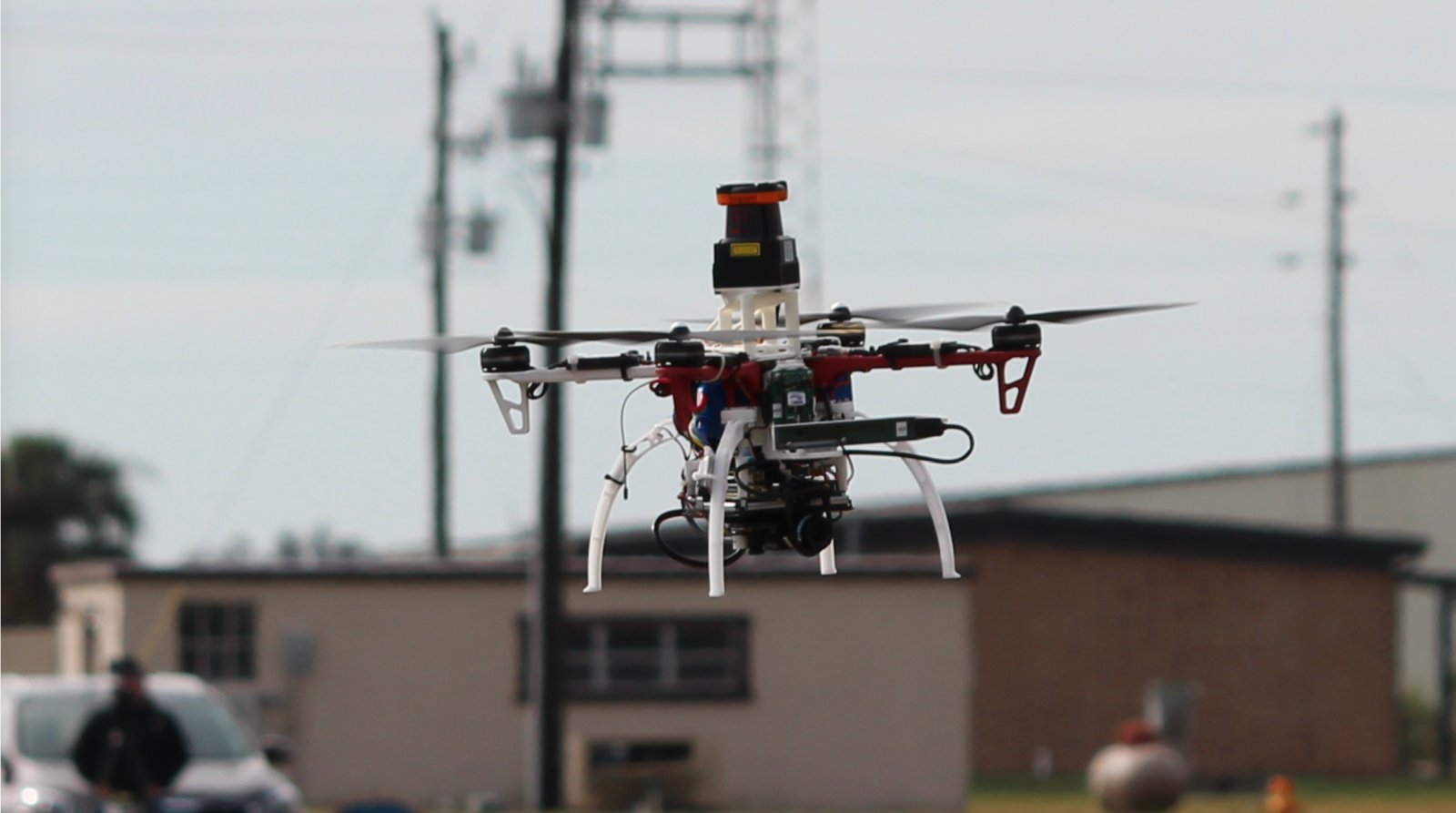
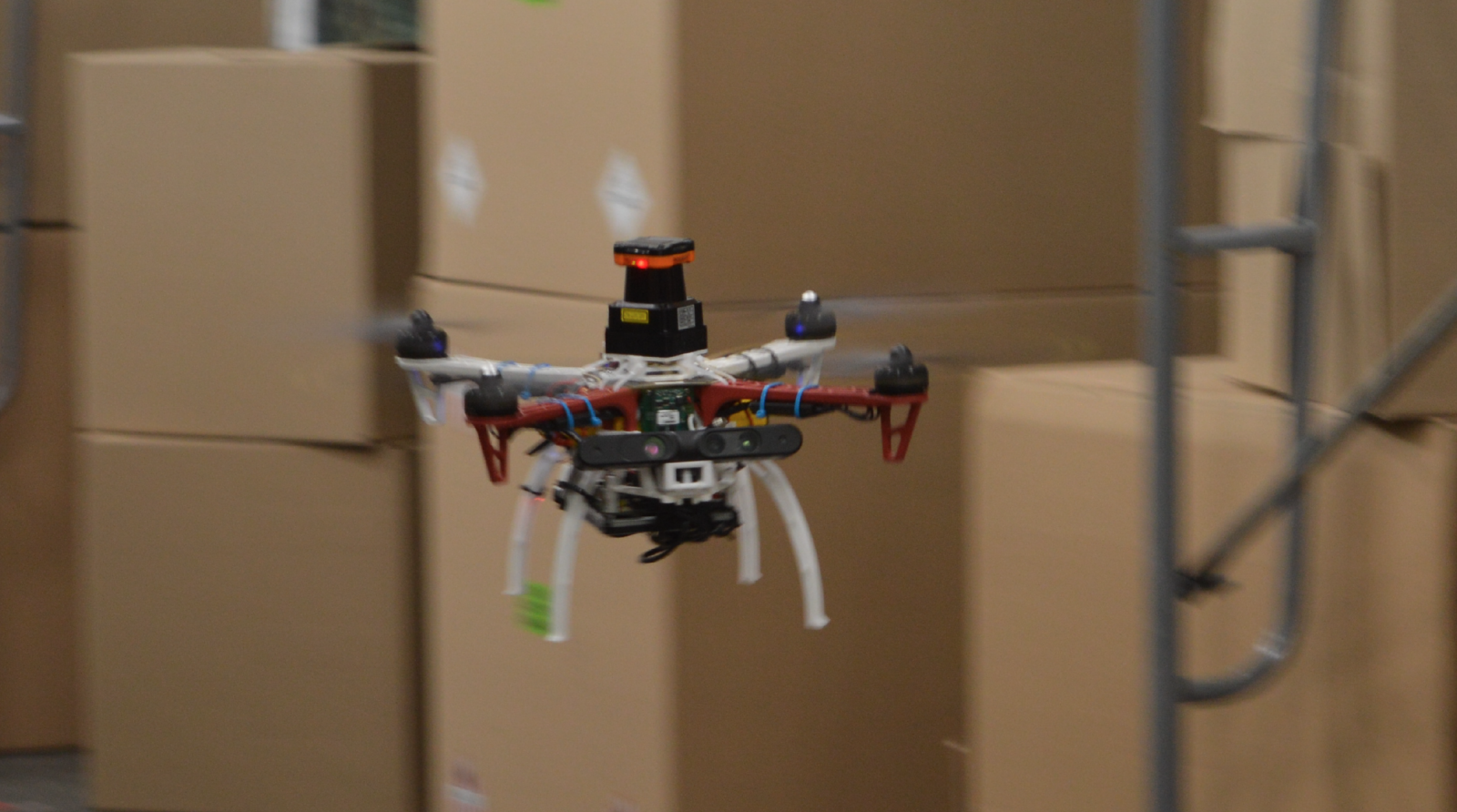
"It's kind of like saving all of the images you've seen of the world as a big tape in your head," says Florence. "For the drone to plan motions, it essentially goes back into time to think individually of all the different places that it was in."
Without NanoMap, the team found that if a drone drifted just 5% away from its target, it would crash one time out of every four flights. When used, however, the coded in uncertainty made sure that the drone crashed only 2% of the time.
NanoMap is able to work well even when placed on relatively small drones that are limited by how much they can carry for real time processing. This is because it does not concentrate too much on the details. It operates by understanding that to avoid an obstacle, the drone does not need hundreds of calculations to place the exact size and shape of what it is trying to avoid. NanoMap simply finds out if there is an obstruction and the general location of where it is.
"The key difference to previous work is that the researchers created a map consisting of a set of images with their position uncertainty rather than just a set of images and their positions and orientation," said Sebastian Scherer, a systems scientist at Carnegie Mellon University's Robotics Institute. "Keeping track of the uncertainty has the advantage of allowing the use of previous images even if the robot doesn't know exactly where it is and allows in improved planning."
A paper detailing their developments will be presented at the International Conference on Robotics and Automation (ICRA), which takes place in May in Brisbane, Australia. This research was supported in part by the US Defense Advanced Research Projects Agency or Darpa's Fast Lightweight Autonomy (FLA) programme, notes the statement.
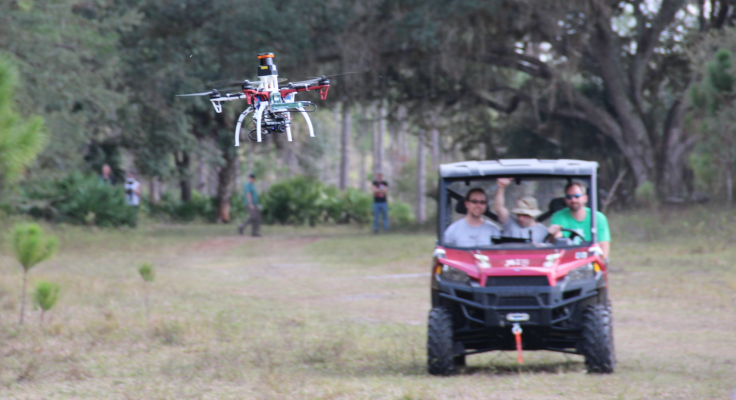