Robotic arm learns intentions of user with breakthrough brain-machine-interface
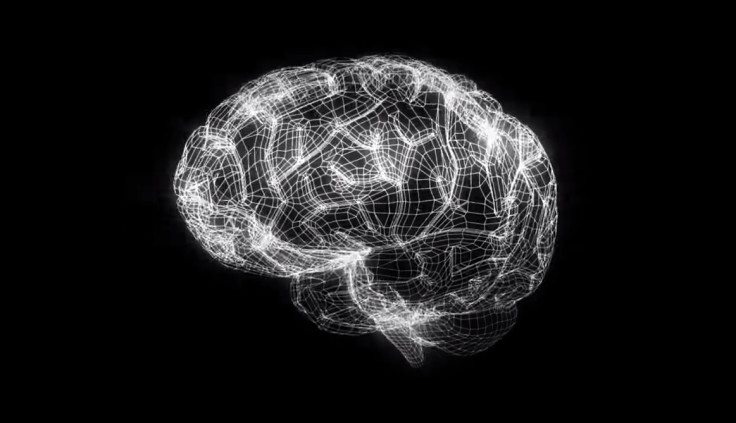
A new generation of prosthetic limbs controlled by the brain is in sight after scientists developed a "self-learning" robotic arm. They could eventually find use with paralysed patients who struggle with the robotic prosthetics that are currently available.
Brain-machine-interface (BMI) prosthetics, known as neuroprosthetics, usually take a long time for the user to be able to send the correct signal in order for the limb to perform the desired task. The latest development allows the device to learn the user's intention in order to carry out complex movements.
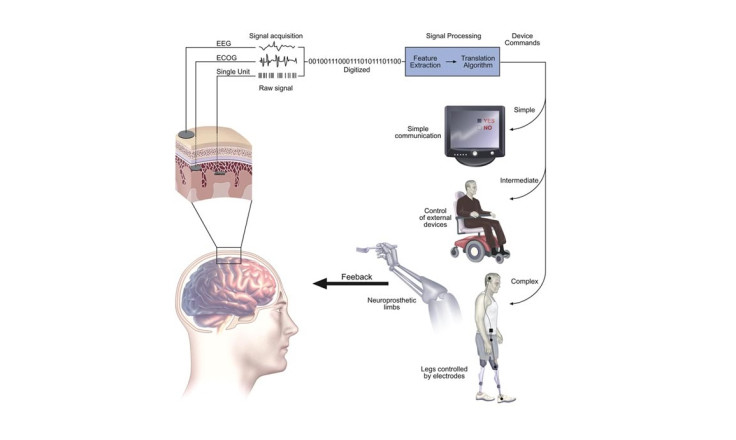
A team of researchers lead by José del R. Millán from the Swiss Federal Institute of Technology (EPFL) developed a new way to utilise an error signal from the brain called Error-related Potential (ErrP) that is emitted when a prosthetic fails in an intended action.
"According to our expectations, this new approach will become a key element of the next generation brain-machine-interfaces that mimic the natural motor control," Millán said. "The prosthetics can function even if it does not have clear information about the target."
During the research, 12 subjects controlled a specific target using a robotic arm placed two metres away. Using the new method, the artificial arm was able to store the correct movements and build an increasing repertoire of appropriate motor actions.
Details of the milestone – described by Millán as a "paradigm shift" – were published today (10 September) in the journal Nature Scientific Reports. "We anticipate this BMI approach to become a key component of any neuroprosthesis that mimics natural motor control," the paper states.
"Furthermore, our paradigm can seamlessly incorporate other cognitive signals and conventional neuroprosthetic approaches, invasive or non-invasive, to enlarge the range and complexity of tasks that can be accomplished."
© Copyright IBTimes 2025. All rights reserved.