How today's machine learning systems would spot another FX or Libor scandal
Tim Estes, CEO, Digital Reasoning, has been invited to talk at IBT's forthcoming data science in capital markets event.
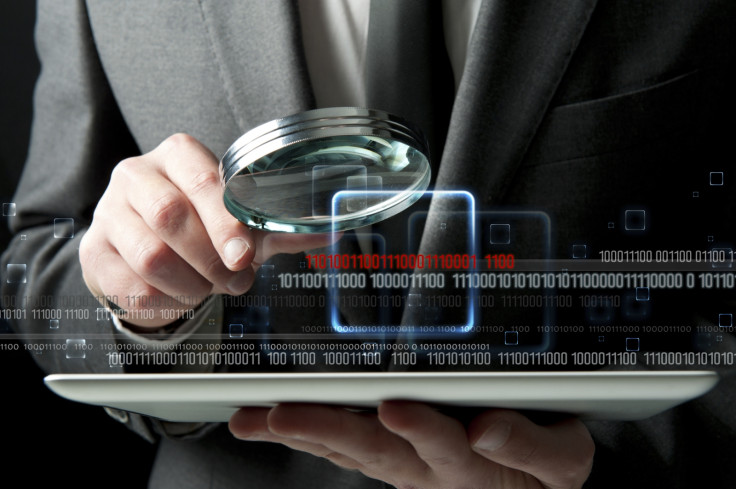
Machine learning surveillance techniques used to spot unfair or collusive activity at firms have advanced far beyond searching lists for risky words, and now make myriad connections based on a wide range of behaviour and interactions.
Tim Estes, CEO, Digital Reasoning, which provides advanced compliance tools of this sort, says that to trick the surveillance software would mean avoiding every system – email, chat rooms etc – and not doing anything behaviourally that would create a signal either.
"If you think about it, it's very hard to be someone that you are not all the time consistently. We have a word for those people – they are called sociopaths," said Estes.
Digital Reasoning is a cognitive computing company focused on applications that leverage human communication data. Beyond the finance sector, where they work with Goldman Sachs and NASDAQ, the company provides large scale health service applications and services some prestigious government contracts (Digital Reasoning runs the largest anti-child sex trafficking system in terms of tools for law enforcement in the US). Banking and finance is one of its fastest growing areas, particularly in light of recent price fixing scandals orchestrated between traders at banks and financial institutions.
Digital Reasoning has honed its technology in the wake of things like the forex scandal uncovered in 2013, where senior currency traders operated in secretive groups and in some cases used coded terminology to carry out manipulation in chat rooms.
Estes said: "FX scandal was a classic case of people thinking that because they were using terminology and very domain specific language that it can't be caught."
In the past this might have been true; legacy surveillance systems tend to rely on lexicons of words that could flag up risk. This can be circumvented by coded terminology, for instance. However, a machine learning system provided with appropriate examples and will start to find patterns, the same way a human would.
"A human learns because they recognise patterns over time," says Estes. "They recognise that the word 'fix' looks a lot like the string f1x; and so just because someone uses f1x in a chat to try to dodge a filter that uses the actual string 'fix', it's not enough anymore, because a computer can see that difference."
"It doesn't mean the technology is as smart as a human. It does mean in some ways that there are patterns human expertise can teach it; and that it can then scale and do something no human can do, which is read one-10m emails a day going through a bank."
Estes is reluctant to talk in too much detail about the nuts and bolts of surveillance, and the ways to potentially make nefarious behaviour more challenging to spot. The short of it is that people have patterns around what they say, where they move, who they interact with, the time they do it, and how they access certain pieces of data.
"Those are unavoidable pieces, they really are. So the question is what pieces show what signs," he said.
Surely the most obvious way to avoid detection would be to communicate on a personal device, possibly outside the office. However, Estes points out that usually the key data needed to act on are locked into these systems. "You can have these various pieces of data in history that are in a system, but you have to stay in that system, like it or not, to actually use it.
"You may have something you perceive as an attachment and there are maybe four ways that you can actually get it moved from an internal system – and of course most of those ways have observations on them."
Map of interactions
Estes explained that Digital Reasoning uses unsupervised machine learning, an assembly of algorithms that cluster and group things from large amounts of data. The machine makes subtle connections, for instance, to recognise that a person's name in one email is the same person being referenced in another mail, even though they are not the recipient or sender. A map of interactions is enriched by an understanding of the context of what's being said. This collated information can then be presented in an easily accessible parcel.
"Humans resolve things based on context and the technology is doing the same thing. It's about accumulating knowledge and evidence in order to build connections and insights and make them more easily accessible to human beings."
"Say I just want to pull up everything about this person who is on my trading floor because they sent this message that really looks problematic – have they conducted any other suspicious activity, have they talked to others, etc.. The pivot from warning to investigation is seamless."
From the perspective of the regulator, Estes said his firm is allowing the bar to be raised. He said everyone knows lexicons and lists of words are not good enough.
"These are terrible systems and have ridiculous amounts of false positives which use up tons of resources and spending for very little yield.
"Feedback from our customers is that the true positive divided by the false positive rate on those kinds of systems flows around 99% – so less than one out of 100 generally is actually a really good hit. By instead leveraging cognitive computing, these same customers are instead reducing their false positive rate by 50-60% and ensuring that the time they do spend investigating directly protects the business."
Newsweek and International Business Times are to host an Artificial Intelligence and Data Science in Capital Markets event, taking place on 1 and 2 March 2017 at the Barbican in the City of London.
© Copyright IBTimes 2025. All rights reserved.