Ashitosh Chitnis: Driving AI & Machine Learning in Enterprise Data Management
Ashitosh Chitnis champions pragmatic AI and machine learning strategies to cut costs, boost compliance, and unlock data-driven value in finance and procurement.
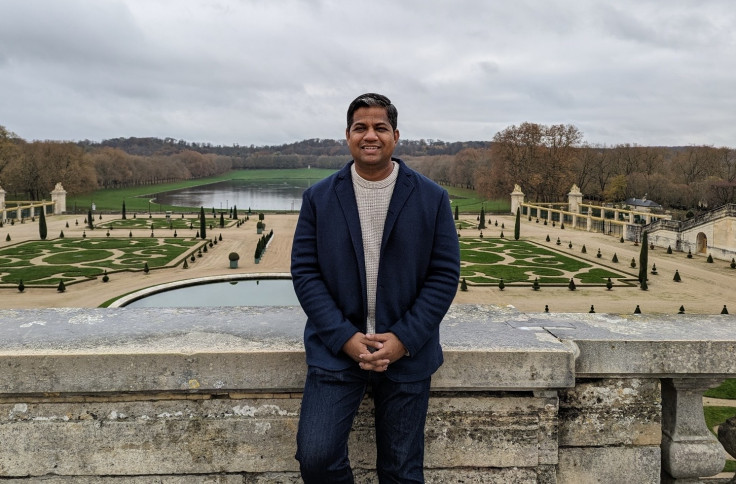
Managing vast enterprise data across complex, often fragmented systems while meeting stringent regulatory demands presents a significant modern challenge.
Artificial intelligence (AI) and machine learning (ML) offer transformative solutions, potentially driving a global AI market that could exceed $1.8 trillion by 2030 and an enterprise AI sector that is forecast to surpass $155 billion in the same timeframe.
With over 80% of organisations already exploring or implementing AI solutions, experienced leaders are crucial.
Ashitosh Chitnis, leveraging over 16 years in data engineering and analytics at technology giants like Google, Apple, IBM, and Deloitte, and expertise with platforms like Vertex AI, guides the practical application of AI/ML to solve core business problems, particularly in finance and procurement.
Taming Data Complexity for AI Success
Data fragmentation remains a primary obstacle for AI, creating inconsistencies and limiting insights. This isn't just inconvenient; data silos impose staggering costs, with one study citing estimates of $3.1 trillion lost annually.
Chitnis tackled this at Google: 'At Google, the finance and procurement teams faced challenges. Traditional rules-based systems were ineffective.'
Unifying data and applying AI yielded substantial results: 'At Google, we leveraged Vertex AI and BigQuery ML, delivering over $50M in annual cost savings. Manual audits were reduced by 90%.' Successfully scaling AI hinges on robust governance.
Chitnis emphasises: 'Establishing and implementing a robust data governance framework are essential. I implemented Master Data Management (MDM) to create a single source of truth for financial and procurement data, ensuring consistency and standardisation across teams.'
AI-driven Transformation in Finance and Procurement
Finance and procurement departments are ideal areas for AI-driven optimisation due to high transaction volumes and stringent accuracy requirements.
Research highlights significant potential for AI to automate numerous finance tasks, driving cost reductions by over 30% in specific functions and fueling adoption, which will reach 58% in finance by 2024.
Realising these benefits necessitates solid data foundations. Chitnis notes, 'I led data engineering efforts to integrate SAP and non-SAP data into BigQuery-based data lakes. Standardising and modelling this data into canonical reporting data marts ensured consistency and usability.'
Continuous effort is vital for sustained value. 'I worked closely with business stakeholders to iterate on models, ensuring they aligned with real-world financial workflows. I also used Vertex AI to monitor model performance, detect drift, and retrain models dynamically,' he explains.
Procurement also sees significant gains; advanced techniques, often AI-powered, can deliver up to 3x higher savings than traditional methods, with strategies targeting 10-15% sourcing cost reductions.
Chitnis stresses principled design and governance: 'A thorough cost-benefit analysis is essential. Explainable AI (XAI) and interpretability are key. Regular drift detection and bias audits should be conducted.'
For compliance, 'To meet SOX and GDPR compliance requirements, it's critical to enforce data lineage tracking and ensure data immutability. Real-time monitoring and anomaly detection guardrails should be integrated.'
Scaling AI Effectively: Technology, Strategy, and Outlook
Successfully deploying and scaling ML models presents significant hurdles; Gartner indicates that only 48% to 53% of AI projects reach production, with failure rates potentially as high as 85%, often due to data or scaling issues.
This underscores the need for robust engineering and operational practices, including proactive data quality management.
Chitnis adds, 'Building automated data quality pipelines is essential to prevent corrupt or incomplete data from impacting AI models. These pipelines should include data validation, cleansing, and anomaly detection checks. Platforms such as Datadog and Grafana are highly effective for anomaly detection and data drift monitoring.'
As AI matures, the focus shifts from it to how organisations can harness it sustainably. Chitnis advises practicality: 'AI projects often fail because they are driven by tech enthusiasm rather than business needs. Instead of experimenting with AI for the sake of AI, start with a problem-first approach—focus on challenges like revenue optimisation, risk mitigation, or process efficiency.'
He stresses foundational elements: 'Ensure data readiness and governance from day one—AI is only as effective as the data it processes. Before deploying AI at scale, enterprises must prioritise investments in data quality, governance frameworks, and scalable architecture. By early implementing AI-driven data pipelines, self-healing systems, and federated governance models, organisations can lay the foundation for sustainable and trustworthy AI growth.'
Enterprises adopting this strategic view are best positioned for future innovation.
The integration of AI and machine learning into enterprise data management holds truly transformative potential. It offers businesses powerful tools to break down persistent data silos, optimise critical financial and procurement processes, and significantly enhance compliance capabilities.
Chitnis's practical experience and insights underscore that realising this vast potential requires more than just technology; it demands a solid data foundation, robust governance structures, effective cross-team collaboration, and the deployment of scalable, explainable AI models tightly aligned with clear business objectives.
Strategic, well-grounded AI adoption is becoming key to competitiveness in an increasingly data-centric world.
© Copyright IBTimes 2025. All rights reserved.