Supercomputers and machine learning used to help identify aggressive brain tumours
Experts hope machine learning algorithms will help to speed up diagnoses.
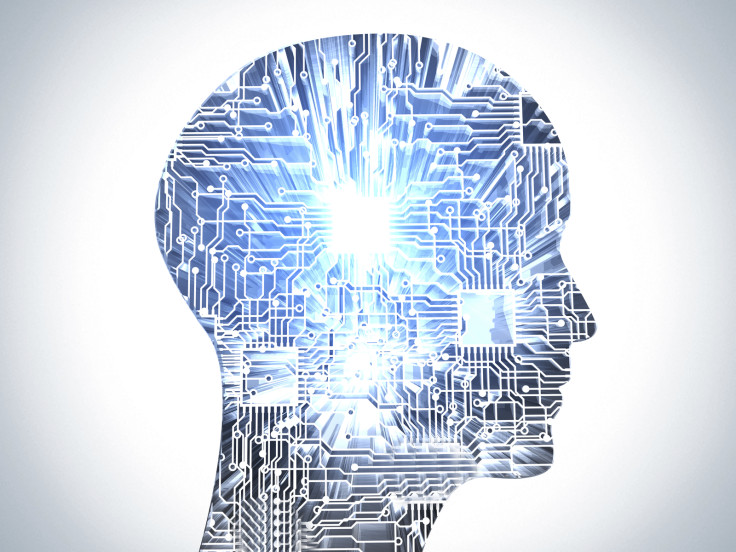
One of the most common and aggressive types of human brain tumour may soon be identified earlier thanks to the use of supercomputers and machine learning technology, according to a team of experts from the University of Texas at Austin.
Currently, specialised radiologists are tasked with accurately characterising tumours – but research suggested that high-performance computing will soon play a supportive role.
George Biros, professor of mechanical engineering at the university, has worked for nearly 10 years to create computer algorithms that can help to automatically scan images to spot gliomas – a formidable type of primary brain tumour.
The technology will not be a substitute for radiologists and surgeons, but experts hope that it could improve assessments and speed up diagnoses.
Alongside a team of collaborators, Biros' team tested their new method during the Multimodal Brain Tumour Segmentation Challenge 2017 (BRaTS 17) – an annual competition where global research groups present cutting-edge computer classification methods.
With the help of supercomputers from the Texas Advanced Computing Centre (TACC), the team's system scored in the top 25% in the challenge.
"The competition is related to the characterisation of abnormal tissue on patients who suffer from glioma tumours, the most prevalent form of primary brain tumour," Biros explained.
"Our goal is to take an image and [...] identify different types of abnormal tissue.
"It's similar to taking a picture of one's family and doing facial recognition to identify each member, but here you do tissue recognition, and all this has to be done automatically."
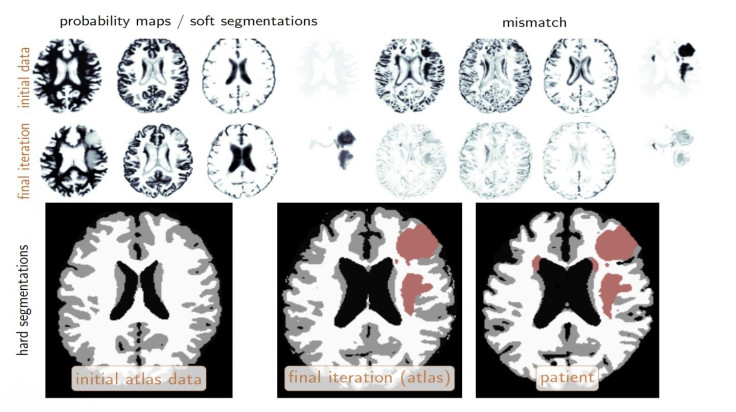
For the challenge, Biros and his team of more than a dozen students and researchers were handed 300 sets of brain images in advance to help "train" their algorithms.
In the test, groups were given data from 140 patients and had to identify the location of tumours and segment them into different tissue types over the course of just two days.
"In that 48-hour window, we needed all the processing power we could get," Biros said.
For every image, the system attempted to determine if an area represented a tumour or a non-tumour. Biros' team ran its analysis in less than four hours and correctly characterised the testing data with nearly 90% accuracy – roughly comparable to human radiologists.
Now, the hope is their methods can be refined, tested and built upon to overtake the abilities of traditional identification techniques.
"We used fast algorithms and approximations to make this possible, but we still needed supercomputers," Biros said.
"We have all the tools and basic ideas, now we polish it and see how we can improve it."
The machine learning system is set to be deployed at the University of Pennsylvania by the end of the year in partnership with his collaborator, Christos Davatzikos, director of the Centre for Biomedical Image Computing and Analytics and a professor of radiology.
Biros said: "This was the first time we put everything together for an application to make our method work for a really challenging problem. It's not easy, but it's very fulfilling."
© Copyright IBTimes 2025. All rights reserved.