Big data-hoarding hedge funds and managing the Twitter 'firehose'
Peter Hafez, chief data scientist at RavenPack, knows about processing large volumes of unstructured data.
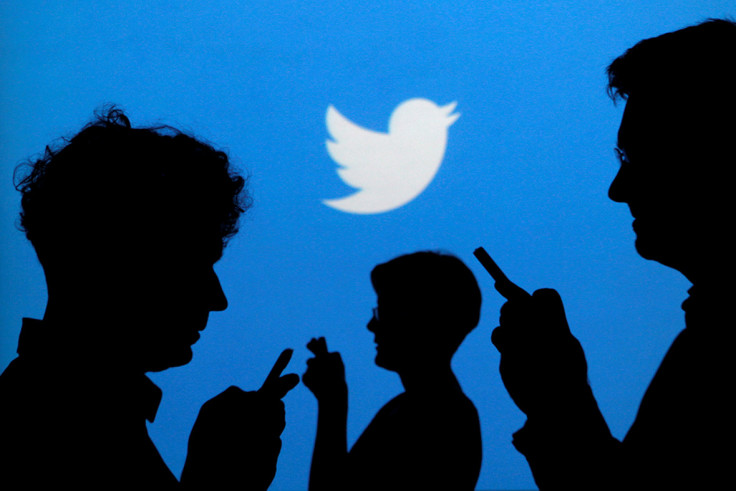
In an effort to beat benchmarks, investment companies sometimes say they are looking at the entire dataset of Twitter, known in the business as the "full firehose".
In actual fact, few people can manage the sheer scale and storage challenges that come with it, not to mention the costs. A hypothesis-driven attempt to do some of this manually is possible but challenging; for instance, you could start searching the social media stream using a hashtag approach.
Peter Hafez, chief data scientist at big data analytics firm RavenPack, knows the market well and how tricky it is to process large volumes of noisy unstructured data. He recounted a story about a small hedge fund, which tried the hashtag approach on "gold", hoping to create a gold sentiment indicator to trade the related futures contracts. Unfortunately, their algorithms didn't take into account how often gold is mentioned during larger sports events like the Olympic Games; and they ended up not being particularly successful.
"Knowing that people's favourite sports athlete had won a gold medal, isn't that useful if you want to measure gold sentiment," said Hafez. "Even though an Olympic gold medal actually does contain some gold, but something like 1.34%. I highly doubt that's enough to move the gold price in any significant way."
Hafez pointed out that taking a whitelist approach might be a better way to go, i.e. identifying for instance a company's official Twitter handle or that of their CEO, of well-known journalists, analysts, economists or opinion-makers. It might still be interesting to search for specific terms or hashtags, say "Brexit", and then see which entities typically turn up when the term is mentioned. In this case, this would have led you to accounts like Boris Johnson's, David Cameron's or Nigel Farage's, and could have provided a strategy to build with. In this case, it might have been interesting to have tracked the sentiment of the "leave" vs. "remain" campaigns to get a glimpse of what the likely outcome of the referendum would have been.
"It's potentially a more discretionary or hybrid type of application, rather than a pure systematic quant approach. However, in the end, you'd still need to rely on text mining to do proper entity detection, noise filtering, and sentiment scoring."
Returning to the question of firms that boast that they trade on Twitter, Hafez said: "besides the ones taking the whitelist approach, most will work either directly with a Twitter sentiment feed, or with a 10% random sample of the full firehose, also known as the 'decahose'."
"Trading on the 'decahose' rather than the 'full firehose' has obvious disadvantages – especially within event trading. You may receive information late on a given event simply because you didn't have access to the entire feed - leaving a potential edge to other investors. However, if you want to do something along the lines of 'wisdom of the crowds', creating a sentiment indicator for a particular company or macro theme, then working with a 10% sample might be a decent proxy."
Several examples exist where events have broken first on Twitter and some CEOs choose this channel first and foremost to deliver news about their companies; a good example is Elon Musk . This leaves data driven players with the challenge of finding the proverbial "needle in a haystack" of signals and noise.
Hafez said: "From a trading perspective, as a whole social media is still considered mostly noise. The technological requirements to provide the necessary trading edge that people are looking for is extremely high. It's already hard to do well within traditional news, and then add the language irregularities of social media on top of that. Not easy! There may be times where social media is breaking news, but we come back to that needle in a haystack type of argument: how often would we have to trade on 'false positives' to get that one 'true positive'."
RavenPack, which today added the UK market news specialist Alliance News to its platform, takes unstructured data, including both news and social media, and structures it on behalf of its clients. The service detects events and sentiment primarily from textual content related to companies, people, places, organisations, currencies, and commodities. For each entity it detects, it also provides relevance and novelty scoring amongst other analytics.
The word on the street is that trading on social media does yield value. However, in contrast to traditional news sources, it lacks an editorial process, which typically leads to lower precision and recall rates as compared to traditional news. "The presence of emoticons, lingo, negation, or the need for tracking user credibility or klout makes it much harder for machines or algorithms to really understand what's going on, and to figure out how it might impact asset prices."
"One of the more promising approaches for solving the credibility issue is to work with a particular whitelist of accounts. You might start out with identifying who are the key players in a given market or company by including the accounts of: big investors, the company's own account, the accounts of their CEO, leading analysts and journalists etc."
"By taking this approach, you might as an investor feel more comfortable with taking an event driven trading approach. However, if you're interested in extracting value from all users across the entire firehose, then from a noise perspective you'll be much more challenged because you are moving into a space that's completely and utterly unstructured."
RavenPack offers some solace to data hoarding financial institutions that have become rather anal about keeping all the data they produce in-house.
This may include all their emails, all their instant messages, all the analyst reports coming from brokers and so on.
"A lot of content, they have to store for compliance reasons, so it's not really an active decision that they make; it's basically a requirement that is often put on top of them. However, given the cost of storing all this information, it's only natural to think about ways to take advantage of it, and to gather further insights from it.
"Let's say I'm a discretionary trader and I'm thinking about buying stocks in Alphabet. I might worry about if there is something in my inbox I haven't read or some discussion on the instant message boards within the firm that talks unfavourably about Alphabet; something that has not been on my radar. That is, is there a contrarian view out there I should take a look at.
"Feeding your e-mail inbox, your brokerage reports, or IMs through an engine like RavenPack could help you identify that contrarian view. That might be one way of addressing this type of data hoarder problem."
© Copyright IBTimes 2025. All rights reserved.