Big data: RavenPack collaborates with Credit Suisse on new Artificial Intelligence Sentiment Index
AIS Index tracks performance of US large-cap sector-rotation strategy, based on sentiment scoring.
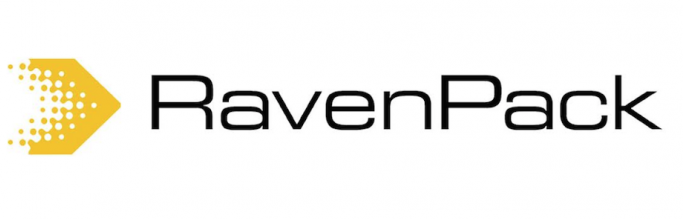
Big data for financial services specialist RavenPack is providing its algorithmic power to Credit Suisse to launch the Artificial Intelligence Sentiment (AIS) Index. The AIS Index tracks the performance of a US large-cap sector-rotation strategy, based on sentiment scoring extracted from news data by RavenPack's artificial intelligence algorithms.
Armando Gonzalez, president and CEO, RavenPack, said there have been a few attempts from companies to create such indices but these have tended to be more like ETFs and other products.
"To my knowledge, there hasn't been anything as high level or as prominent where there is an actual bank that is looking to deploy this type of index; there is definitely novelty in the grandeur of the application, where before it has only been tried mostly in the social media space and perhaps more from a marketing standpoint," said Gonzalez.
RavenPack concentrates primarily on newswire services, national, regional and local newspapers, as well as professional services feeds and blogs: a carefully curated list of about 19,000 plus sources form the input.
"Algorithms decide which sources at what point, for what content, should serve as the factors that go into these quantitative investment strategies," said Gonzalez. "At the end of the day, it's an aggregate view of sentiment across thousands of potential constituents that effectively serves as the sentiment factor.
"So it's not just one particular source; it's an aggregate view of the messages and the sentiment across news stories and blogs and other types of documents."
Twitter is not one of RavenPack's socials; regarding the usefulness of Twitter for professional investing, Gonzalez said: "There are definitely different opinions. But when you compare the academic and the professional sell-side research on both newswires and professional media versus Twitter, you will find a predominant inclination towards news."
"There is quite a significant gap between what's been found in research on professional news wires and on filings and on earnings transcripts and earnings press releases etc., versus the opinions that are expressed in Twitter.
Some of the key findings Gonzalez alludes to are things like limited coverage. Twitter perhaps offers opinions on some of the larger cap companies, and those that are more media prone, such as Apple and Google and Microsoft, but fails to cover mid-caps and small caps.
"It's actually quite rare in fact that you will get 'noise free' information from Twitter. And if you do, there is always the risk of market manipulation or there are biases and the sample size is just too small.
Gonzalez says Twitter tends to become more of a source for boutique investment shops, or for smaller operations, or for niche strategies which focus on the tech sector or consumer retail for example.
In addition to the problem of scaling, another big challenge is the sheer noise. "It's essentially a very noisy environment that you are working from. We have decided not to get into that because we don't believe that the state of the art of AI is there to be able to deal with fake news or deal with that level of noise or intentional manipulation. The huge amount of noise in Twitter makes it impossible for algorithms to really understand what's going on.
"I would also say there isn't enough historical information yet. With news you have digital archives spanning 20 years, even 30 years in some cases. You can go back to economic cycles, you can go back to the dotcom bust, you can go back to the financial crisis, or the European credit crisis, and see how markets reacted. With Twitter you are very limited to some of these cycles; you end up finding one off flukes, as opposed to real trends."
Essentially RavenPack's AI is a stack with a number of different modules that take care of specialised tasks and are extremely good at identifying entities. Providing a high level view of what the algorithms do, Gonzalez said: "So we have a unique identifier for the company Apple, in the same way as we have a unique identifier for the fruit apple. The algorithm is competing against other algorithms to determine whether references and text to the actual company are legitimate."
The algorithms need to know the entities to produce analytics like sentiment accurately. If a story talks about multiple companies, it may contain multiple sentiments. For example, it could be highly bullish on Apple, but bearish on Microsoft; or it could be passively talking about Tesla, but really be about Alphabet.
"All these different distinctions have to be made by the algorithms in a very precise manner. We tell how relevant these entities are in every document and then we do semantic analysis and figure out from what context are these entities being referenced," said Gonzalez.
"We can tell if a company is involved in a lawsuit; we can tell if the company is the plaintiff versus the one that is the defendant. If the company is involved in an acquisition, we can tell which company is the target, versus the one that is the acquirer.
"We have hundreds of these well-defined roles that companies play, and in doing so we can provide a taxonomy for clients to understand the information in a more systematic way. Everything is highly predictable so they can see and build rules around the thing that the algorithms know how to detect.
"So, we are a very pragmatic artificial intelligence company. We don't tend to romanticise AI; it's a tool and it's there to help us solve specific problems: to try to identify new information around a stock or a country or a commodity that isn't already incorporated or available in other fundamental or market data – which is what the majority of investment managers use today."
Armando Gonzalez will be talking about big data at Newsweek's AI and Data Science in Capital Markets conference on December 5-7 in New York, the most important gathering of experts in artificial intelligence and machine learning in trading. Join us for three days of talks, workshops and networking sessions with key industry players.
© Copyright IBTimes 2025. All rights reserved.