Credit Suisse charts the evolution of artificial intelligence and investing
The distribution of AI-enabled research is seen across three classes of adopters: advanced trading, competitive data scientists and master data scientists.
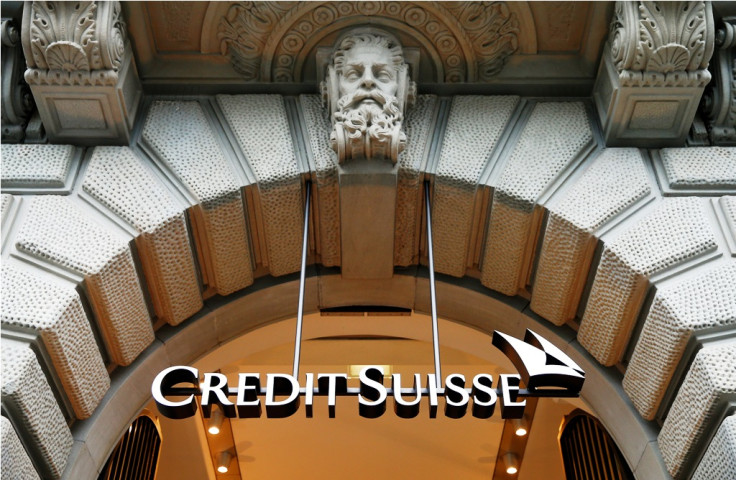
"We are currently experiencing one of the most significant periods of technological advancement since the early 1800s industrial revolution," says Ekaterina Sirotyuk, Portfolio Manager, Credit Suisse. "This is bringing waves of change across industries, and the world of finance is no exception."
However, Sirotyuk sees this more in terms of evolution rather than disruption. She cites the rise of index-based investing; back in the 1980s these sorts of funds may have charged an upfront fee of 6%, whereas now you're charged 0.05% for many index-based solutions.
For many years now, investment firms have used ever more advanced computing techniques to their advantage in the analysis and interpretation of data. But even the most advanced techniques were often constrained by processing power, used limited data-sets, and relied on inhibited human thinking to define an approach.
"Today, due to the technological advancements of recent years, the financial industry need no longer be held back by these constraints, and is starting to use artificial intelligence in a more fundamental way," said Sirotyuk.
"Previously when we referred to enhanced quantitative systems we spoke about quantitative investing, whereas now we see how data availability and advanced computing techniques make the investment process across a number of strategies much more data driven."
Sirotyuk and her liquid alternatives team at Credit Suisse have considered a changing spectrum of data, from structured data (price and fundamental data) to unstructured data, including text to numerical data, big data from satellites and so on. The distribution of AI-enabled research is seen across three classes of adopters: advanced trading, competitive data scientists and master data scientists.
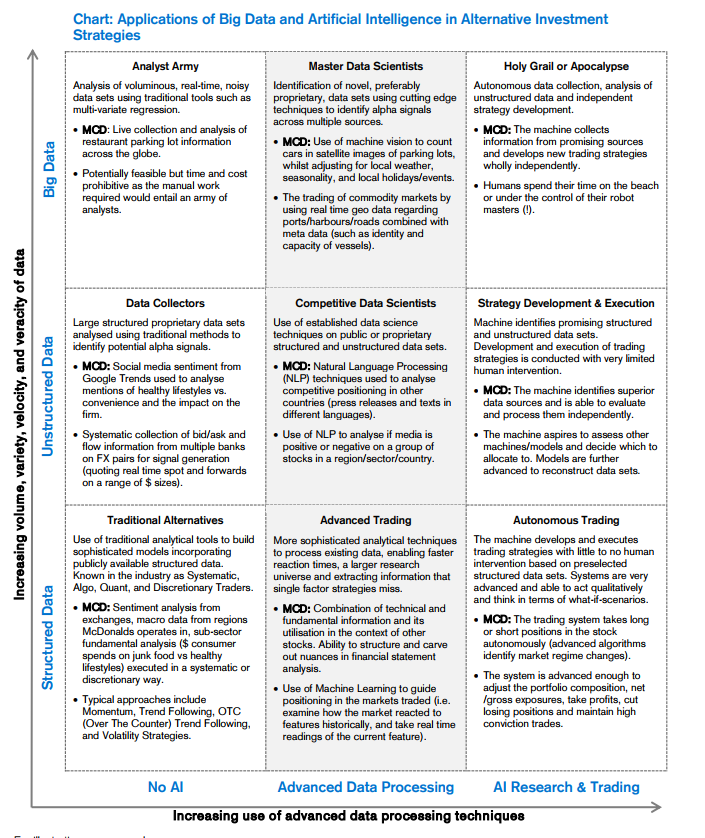
"What we classify as advanced trading, this uses sophisticated analytical techniques to process existing data and enable faster reaction times. They are able to process large data sets or text and extract valuable information. A good example is where you have different footnotes in balance sheets or other company statements and you are able to pick it up systematically.
"When we speak about competitive data scientists, we talk about people who use public and proprietary, structured and unstructured data sets. For example, a portfolio manager who would try to use natural language processing (NLP) techniques to analyse if media is positive or negative on a group of stocks in a region or country.
"Finally master data scientists are the ones who probably already use things like very advanced satellite images to understand the position of vessels on the sea or in harbours, in order to understand the flows in the market.
"As time passes we would expect to see people going from structured data and no artificial intelligence to incorporating some elements of less structured data and more advanced data processing techniques. The way they do it will obviously depend on their skill set, as well as the availability of certain data or developing their own proprietary data sets."
Returning to the evolution of passive investing and smart beta, is it possible to justify the costs involved in using lots of technology to crunch through large datasets?
Sirotyuk said: "It's true that data can cost, but that's not always the case; if you're an established firm you might have been producing data or assembling data as part of your existing process. So, you just systemise it as you handle it over time and we see examples of this.
"Firms that roll out in independent strategies have typically done quite a lot of research before. They have tried to figure out ways in which algorithms can be complementary to what they already have, such as momentum, which is a very common reference, as well as other quant macro strategies."
There's a large amount of hype out there; when someone is touting multiple years of back-tested AI and big data, "I take it with a pinch of salt," said Sirotyuk.
"Some of the data became available really quite recently and it's tied up in terms of how to pay, store, process, and compute on it. It doesn't mean that elements of machine learning per se have not been used; they probably have.
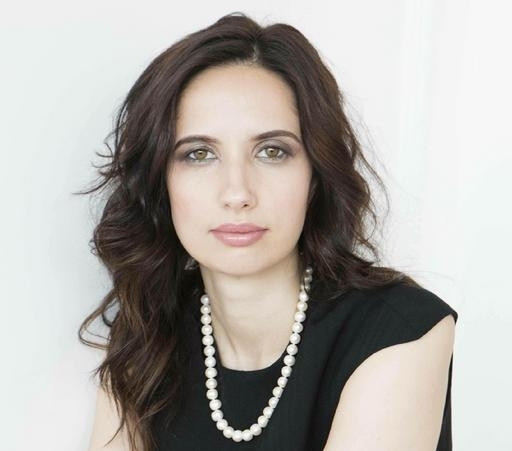
"Also, many big investment firms have been using a lot of neural networks in execution for some years now. If you have different orders coming through the day and you don't have a linear function for how to execute that, then you have to figure out which way you scale your execution. Neural nets are especially good at finding those solutions.
"Ultimately you can argue that increases your post trade alpha," she said.
Ryan Bennett, Alternatives Advisor at Credit Suisse, who works with Sirotyuk's team, said lots of requests for information about AI investing have been coming from the likes of family office clients. Firms are looking for guidance amid all the hype, he said, which involves a different approach to what they might be used to.
"Even some of the more sophisticated clients that we deal with want to look an investment expert in the whites of the eyes in order to feel confident about the skill and experience they've had across the industry and the returns that they have generated," said Bennett.
"When it comes to assessing the skill of an algorithm or the completeness of a dataset different techniques are required."
He sees a lot of interest is coming from entrepreneurial clients, who may themselves have been disrupters within their own industries.
"We often have discussions with the next generation of clients, people taking over portfolios or who have inherited a portfolio. They are looking at it with a fresh set of eyes and are interested in going into areas which are perhaps more innovative and dynamic.
"They are also coming from our entrepreneurial client base; they tend to have been first movers within their respective industries and understand that being innovative can lead to big opportunities," he said.
Newsweek's AI and Data Science in Capital Markets conference on December 6-7 in New York is the most important gathering of experts in Artificial Intelligence and Machine Learning in trading. Join us for two days of talks, workshops and networking sessions with key industry players.