Developing pharmaceuticals efficiently with artificial intelligence
Ken Mulvany, Founder and Chairman of BenevolentAI, predicts as much as 60% time saving in early drug development phases compared to traditional pharmaceutical industry averages.
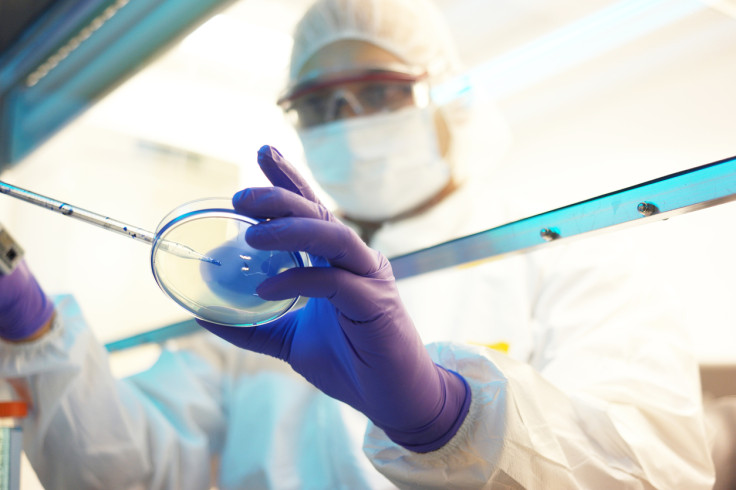
Computing has evolved explosively, particularly in the last decade, bringing benefits to a broad range of fields. At the same time, so has the data being generated - 90% of the world's data was created in the last two years, far more than we could ever humanly comprehend. Yet, interpreting certain data is critical to advancing science. Developing analytic methods that can make sense of the vast quantity of data is the key to unlocking understanding of this buried knowledge.
One such field of science is pharmaceuticals. The process from identifying a new molecule to it getting to market as an approved drug, takes between 15 and 20 years, even with recent advances in technology. What's more, the overall success rate to develop a new drug remains extremely low at around 5%, and can cost anywhere up to $2 billion per new medicine.
That is an unsustainable rate of failure, particularly as the industry moves from the era of blockbuster medicines to personalised medicine. Without action, the successful identification of new candidates will continue to decline. To ensure that the industry continues to develop lifesaving treatments we need to find solutions that create efficiency in the drug discovery process.
How can AI revolutionise the drug development process?
It is estimated that the average academic researcher reads between 250 and 400 articles per year. While we now have vast library of knowledge regarding disease pathogenesis, with 50 million scientific papers available globally and thousands added every month, to analyse each and every piece of information on each biomarker that exists, is humanly impossible.
What is required is a way to improve the efficiency of target selection, optimising compounds and drawing previously unachievable insights from the millions of associations between entities and unstructured texts. Whilst researchers specialise in their own particular field, they may only ever read around their own specialisation, leaving little possibility to create connections with other fields. There are a number of diseases that may be the result of numerous pathological and physiological factors that may have been discovered across specialties but have not been connected. Alzheimer's disease, for example, is considered the outcome of an interplay between immunological and neurological factors.
By analysing considerably more information than is humanly possible, AI can help researchers correlate, assimilate and connect data, structuring this through the help of algorithms and annotations. This process of structuring relevant information from various sources enables researchers to delve deeper into the information, at an accelerated rate. By bringing this information together, connections are made that would not have been made under normal circumstances.
What does that promise mean in reality?
The often pain-staking process of drug target identification, and the process of moving to in-vitro or even clinical testing is improved in terms of efficiency. At BenevolentAI, we have predicted there to be as much as 60% time saving in early drug development phases compared to traditional pharmaceutical industry averages.
The promise of efficiency in the development process has shone through in the treatment developments for Amyotrophic Lateral Sclerosis (ALS). The AI platform, developed internally, was able to analyse billions of sentences and paragraphs from millions of papers and abstracts, bringing together relationships and direct links within that data, which were then termed as 'known facts'. This information was then curated so that unknown connections can be made – generating previously unrealised treatments from peer-reviewed and approved scientific papers.
Despite no technological or scientific expertise in ALS, in just half a day BenevolentAI's technology produced a list of potential treatments which were then triaged and tested by BenevolentAI's scientists. After a week the five best potential treatments for the disease were taken to a world authority on ALS – the Sheffield Institute for Translational Neuroscience (SITraN).
They were astonished by what BenevolentAI had done. One of the treatments matched their ongoing research and two were completely novel - ideas they later admitted they would probably have overlooked had BenevolentAI not come to them. The company and SITraN started to work together to take a drug candidate forward. In May 2017 SITraN announced after a year of study and testing that the work, the invention proposed by BenevolentAI's machine, had delivered positive results.
In fact, the research showed that the machine may have found a way to prevent the death of motor neurones - the key to finding a cure for this disease – it's was a huge inventive step. essentially a big bang moment for medicine. A point where, instead the dystopian prophecy that machines will ending humanity, a machine began to try and cure humanity.
What else is in store?
While initially developed to understand the underlying cause of disease and propose treatments, the AI platform is able to explore other R&D processes. This includes predicting the properties of chemical compounds, reducing the number of other complementary compounds to be synthesised, and consequently enriching the 'chemical palette' available to medicinal chemists. In addition, it excels in identifying adverse effects and safety issues of these compounds.
Beyond the research and development stage, AI's ability to access and analyse diverse datasets can also bring opportunities when designing clinical trials. This is a lengthy process for any drug maker, consisting of a sizeable amount of information to be analysed before it can be put into motion. Hence, AI provides the opportunity to extract new insights into how a certain patient might respond to treatment and to help organisations recruit patients for trials. Beyond this, AI also has the potential to speed up the process of analysis of medical imaging scans, reducing the workload for clinicians.
Reinvigorate not Replace
Whilst AI does not present a replacement to the researchers behind the drug discovery process, it aims to build upon and take full advantage of the vast of knowledge they have gathered. The human challenges that are continuously faced in the drug discovery process have limited the rate at which disease treatment developments can be made. AI works to ultimately reduce the time and errors that occur throughout this process, thus mediating the 5% success rate. By acting as a catalyst to human-based research, AI presents a game-changing way to evolve healthcare across its numerous steps, from clinical research, to diagnosis, to treatment.