How hedge funds can trade on heaven-sent data
Day 1: IBT Media's data science and finance event looked at alternative data sets and natural language processing.
Delegates at Newsweek and International Business Times' data science in capital markets event were mesmerised by a video of shoe box-sized satellites, known as "cube sats" being released into the earth's atmosphere. They were then shown the speeded up footage that was being captured: giant oil tanks with floating lids, rising like tides; ships being built from scratch in dry docks; burning flares from steel mills; a picture of the agricultural yields of the entire planet.
Professor David Hand, chief scientific advisor, Winton, introducing the event, pointed out that the current AI summer is characterised by what he called "automatic data capture".
He said: "Perhaps even more important than big data, we can now observe what people are doing all the time, like shopping for instance. Heterogeneous data is being produced constantly, as you sit here data about you is being captured by computers."
The satellite images came courtesy of A.J. DeRosa, executive VP, Global Insight. "Moore's Law is going to space," he stated. Rather like the reduced cost of computers, satellites have also shrunk in cost and size, from $750m space craft the size of buses to the handy cube sats ($100,000), which are now permitted for use in any sort of commercial enterprise.
"Imagine being able to count ships at port," said DeRosa. "Imagine being able to count anything. Skynet is here! Without GPUs none of this is possible."
In terms of creating value from a trading and investment strategy, he said crude oil tanks filling and emptying has an obvious direct bearing on people interested in commodities. "Want to measure oil in China? First you need to know where the oil tanks are. The Chinese don't like to share this information. There are publicly known to be 500 tanks; we counted 2,000.
"We can look at the entire planet for the first time. And satellites have been around for a while so there is historic data so you can back test."
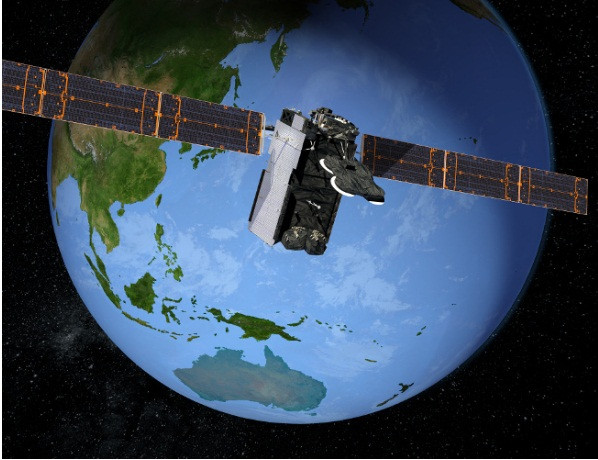
The explosion in alternative data has given rise to a new secondary market where hedge funds are connected to interesting and unusual datasets which their competitors may not have access to. Tammer Kamel, CEO, Quandl, is a data specialist who understands the transient nature of alpha-generating advantages. We saw this with the first uses of computers to price bonds which made a fortune for some because the rest of the street was using calculators, he said. More recently we have seen the same sort of thing with HFT.
"You can just go out into the wilderness and find data. It's a gold rush. Anything you want to know about the macro economy can be known today if you are connected to the right database," said Kamel.
He said many organisations are sitting on interesting data sets that the market doesn't know about. For example, the insurance company which has a list of the policies sold on new cars. Not only is it a measure of new car sales, it can be broken down further into manufacturer, like new policies on BMWs for instance.
Another Quandl example uses automatic identification system (AIS) which maps the whereabouts of all vessels on the ocean via inbuilt transponders. "It's an awful hairy dataset but it tells you where all the ships are in the world. Then you can add a second proprietary dataset about ports and berths; what do they load there, oil, coal, whatever. Mash it up with AIS data and you can work out how much coal went to China this week."
Kamel said another revealing dataset can be constructed from various suppliers and service providers to find out which companies can pay their bills on time – with a view to potentially shorting their stock.
Leigh Drogen, CEO, Estimize agreed that alternative data is a fine thing, but how do you get from signal to strategy. He said the discretionary world is clueless when it comes to data science. He recounted how a discretionary portfolio manager with billions under management came and asked if he could be taught the rudiments of data science. "I just don't have 12 nights out of my life to teach a class," said Drogen.
There are good ways and bad ways to go about integrating data science into investment firms, noted Drogen, castigating the centralised PM or pod-based approach; complete collaboration is a better way to understand what these quantitative findings really mean to your strategy. "Put a quant on the desk, and usually an engineer too," he said.
John Macpherson, CEO, BMLL, a startup which uses machine learning on limit order book data, said common RESTful APIs, the cloud and in general the "plumbing" is crucial. "You want the data to arrive in the right format. Data scientists spend most of their time massaging data. They should really be called 'data janitors'," he said.
After lunch the focus turned from data which is mainly numerical in origin on to text. There has been a lot of work done on natural language processing and sentiment analysis – and the challenges involved have only been exacerbated by the addition of social media.
Gideon Mann, head of data science, Bloomberg, explained that algorithms learn to deal with things like changes in the ways companies are referred to, such as Bank of America or BoA, or BoA Inc. Social media added to the mix means yet more ambiguity because it has its own style.
Mann touched on the problem of fake news which he compared to email spam or news spam. He said algorithms were probably the solution. "A tweet came from a particular place; what router, phone, laptop. This is not the be all and end all regarding veracity measures with Twitter, but it is one way our thinking is going."
Edin Zajmovic, director of investment management – EMEA, Thomson-Reuters, began at the beginning, when Reuters employed carrier pigeons to transmit closing prices between London and Paris. Today he is using deep learning to create "the largest graph of financial data bringing in structured and unstructured data". This system becomes comprehensive as it grows, using deep learning to link companies that may be impacted by certain news stories.
Peter Hafez, chief date scientist, RavenPack pointed out that they beat Thomson-Reuters by six months to bring out the first sentiment product. Hafez talked about providing treatment for hedge funds' data hoarding habits – a common disorder around a fear of discarding anything.
"One discretionary trader came to me with 20,000 unread emails. He was worried that when he was about to trade something, there could be information in his inbox which would give him a contrarian view," said Hafez.
Peter Bailey, chief strategy officer, Dataminr, which looks for patterns amid tweeting within the full Twitter "firehose" said he thinks of it as a trip wire that's 320 million users long and 50 languages deep. This is a needle in a haystack problem, but often things like oil pipeline explosions which quickly become the subject of localised tweeting, open windows of arbitrage advantage.
Asked for predictions about what sort of data will be the most interesting in the future, Bloomberg's Mann said he is a language guy. But looking at AI in general, he wondered about the effect of self-driving trucks, for example, which in a few years' time will put about eight million people out of a job. "That's just trucks," he said.
Bringing it back to investment strategies, the brilliant Michael Beal, CEO, Data Capital Management, had a stark prediction: "If you're finding alpha then you will be fine. But if you package beta, it's about to be over for you."
© Copyright IBTimes 2025. All rights reserved.