Machine learning: Taming the flood of unstructured enterprise data
Matt Fleckenstein, Nintex CMO, discusses how machine learning can extract value from an ocean of unstructured data.
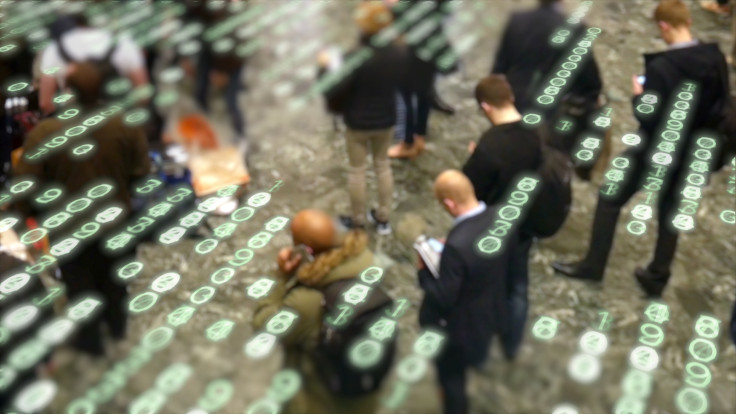
Enterprises are facing a paradox. They know that today's hyper-competitive business conditions require them to make key decisions based on data, not just intuition. They're working toward this goal by capturing vastly more operating information than ever before. The problem is that much of this data is of little value because it is unstructured and can't be analyzed by conventional means. However, two emerging technologies – machine learning and intelligent process automation -- can now be harnessed in powerful solutions that deliver insight, drive smarter decisions, and help businesses become more agile.
Businesses have always relied on "structured" data" -- spreadsheets, forms, HTML pages, and the like – to inform their plans, strategies, and decisions. They still do. But the fastest-growing types of data today are things like emails, blog posts, tweets, texts, images, log files, sound files, and chatbot conversations that lack traditional structures, and this unstructured data is, in essence, opaque.
Structured data has been managed by means of metadata (data about the underlying data) and elaborate rules for sorting, categorizing, storing, and retrieving it. But unstructured data defies this approach. You simply can't write enough rules to adequately describe all the potential attributes of the flow of emails in an organization, for instance, or all the images that you might need to track. As a result, all of this unstructured data is extremely difficult to analyze. Enterprises store much of it (and spend increasing amounts to do so) because they know it must have value, but they can't see into it, so they haven't been able to unlock much of its value.
Research firm IDC estimates that digital systems by 2020 will have generated some 13,000 exabytes (roughly equivalent to the complete works of Shakespeare, digitized, then multiplied by a million, then by a billion). One-third of this data would have business value, IDC says, if there were some way to analyze it – and then to do something with the analysis.
Fortunately, the era of opaque unstructured data is finally ending, thanks to rapid progress in machine learning and intelligent process automation. Together, these two disciplines give us the means to extract and apply the meaning inherent in the massive clouds of unstructured data enterprises are capturing.
Machine learning relies on algorithms that can analyze vast amounts of data to hunt for patterns and can be trained to form progressively smarter "hunches" about the meaning of what they find. The oft-cited example is an algorithm that accurately finds all the cats in a massive database of random images, not by following deterministic rules but by asking, in essence, "I think this is a cat – am I right?" and using the results to refine its "idea" of a cat.
In terms of business documents, an algorithm could be trained to parse complex documents and distinguish between those that are completely standard and those with important exceptions, such as a deeper sales discount than allowed by company policy. At that point, we can use intelligent process automation to approve the standard documents without human review, while routing those with exceptions to the appropriate person based on conditions such as subject matter expertise or availability. For example, the system could be instructed to minimize approval time and then trained to learn the conditions that govern approvals, such as whether an office is closed or whether the appropriate person is on leave.
Today, both machine learning and intelligent process automation are available as cloud-based services that make them easy to adopt, tailor for specific situations, and modify based on how well they perform. In a typical business process, each step might involve a different type of unstructured data and thus require a different type of machine learning – image recognition to authenticate users, natural-language processing to parse the meaning of an email thread, automatic generation of custom documents tailored according to the user and the email content, and so on. When these capabilities are made available as services, they can be invoked without writing any software code.
This type of solution architecture has several advantages. One is that solutions need not be designed and implemented by software developers. They can be built by the people closest to the processes involved, the people best positioned to identify the critical paths and bottlenecks the process must address. These same people are also best positioned to judge whether an automated process is working as intended and where it might be improved.
A crucial point is that automated processes, because they are easy to create, are also easy to modify and improve, for example by invoking a different type of machine learning, by changing the approval path of a document, or by addressing a limitation that became evident only with experience. With the right tools, it's a straightforward task to automate, analyze, and optimize key processes.
Such solutions are exceptionally powerful because there is synergy across machine intelligence, process intelligence, and human intelligence. Machine learning algorithms become steadily smarter in finding the meaning formerly hidden in opaque unstructured data. Automated processes become smarter and more effective as people apply their experience and judgment to each iteration.
By harnessing these types of intelligence together, businesses can bring to light all the insights in the mounds of unstructured data they now have at their disposal, and put those insights to use in smart, automated workflows.
Matt Fleckenstein is the CMO at Nintex, a recognized global leader in Workflow and Content Automation (WCA). He brings 20 years of technology experience to Nintex and previously worked in the artificial intelligence marketing technology sector.