MIT develops system that can detect 85% of cyberattacks using artificial intelligence
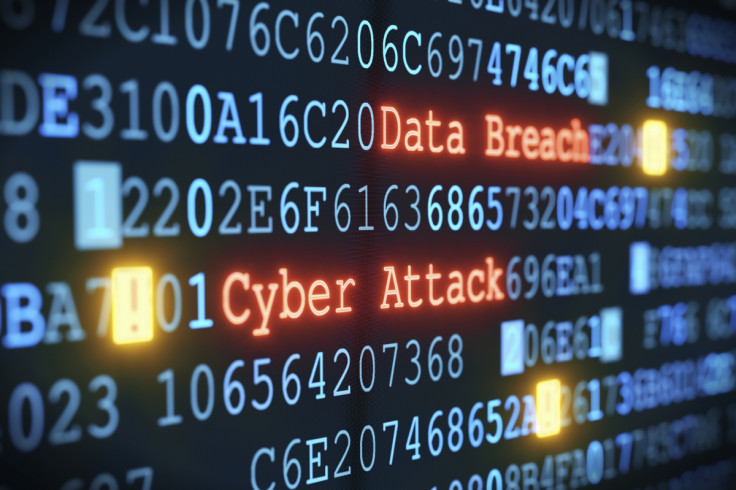
Computer scientists from MIT and a machine learning startup, PatternEx, have reportedly developed a new system that can correctly detect 85% of cyberattacks using artificial intelligence merged with input from human experts.
At the moment, security systems are closely monitored by humans and programmed to pick up on cyberattacks that only follow very specific rules, as such missing any attacks that do not follow those rules.
But, there are also systems autonomously run by computers that practice anomaly detection – i.e. the identification of items, events or observations – that do not conform to an expected pattern or other items in a dataset. This method often leads to false positives, meaning that humans doubt the reliability of the system and are forced to go back and check all the results anyway.
To improve this, researchers from MIT's Computer Science and Artificial Intelligence Laboratory (CSAIL), in collaboration with PatternEx, have developed the AI<sup>2 artificial intelligent platform, which merges three different machine learning methods that enable computers to learn unsupervised.
Rather than requiring cybersecurity analysts to spend all day analysing huge amounts of data that may or may not be a sign that cybercriminals are attacking a network, AI<sup>2 is instead trained to pick out the 200 most abnormal events it has detected during that day.
The human expert looks at the events and picks out which events relate to a cyberattack, and as days pass by, the computer learns how to identify more and more of the events as attacks, accurately, by itself – meaning that, eventually, the cybersecurity analyst might only need to look at 30-40 flagged events per day.
"You can think about the system as a virtual analyst," said Kalyan Veeramachaneni, a research scientist at CSAIL who developed AI<sup>2 with Ignacio Arnaldo, a chief data scientist at PatternEx and a former CSAIL postdoc.
"It continuously generates new models that it can refine in as little as a few hours, meaning it can improve its detection rates significantly and rapidly."
When tested using 3.6 billion pieces of data called "log lines" that were generated by millions of users over three months, AI<sup>2 was able to correctly identify cyberattacks roughly about three times better than previous systems, while also reducing the number of false positive results by a factor of five.
"The more attacks the system detects, the more analyst feedback it receives, which, in turn, improves the accuracy of future predictions," said Veeramachaneni. "That human-machine interaction creates a beautiful, cascading effect."
The paper, entitled "AI<sup>2: Training a big data machine to defend" was presented at the IEEE International Conference on Big Data Security on Cloud (BigDataSecurity 2016), held in New York from 8-10 April.
© Copyright IBTimes 2025. All rights reserved.