Former nuclear physicist Henri Waelbroeck explains how machine learning mitigates high frequency trading
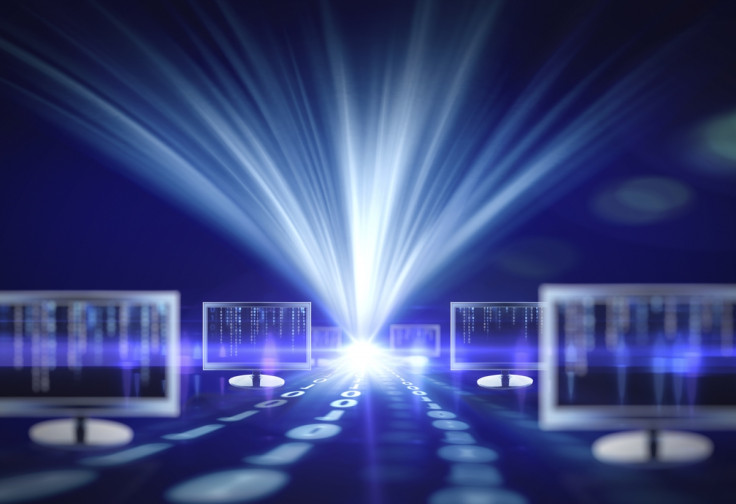
Henri Waelbroeck seems to fit the popular image of the scientist transplanted into the world of high finance and hedge fund trading, the sort of stereotype found in books like "The Fear Index" by Robert Harris.
Waelbroeck, director of research at machine learning-enhanced trade execution system Portware, was previously a professor at the Institute of Nuclear Sciences at the National University of Mexico (UNAM). His areas of expertise include: complex systems science, quantum gravity theories, genetic algorithms, artificial neural networks, chaos theory.
The impression Waelbroeck conveys is one of precision. He explains that algorithms have grown in complexity since being introduced to the world of trading around 2000. This has made it increasingly difficult for traders to understand each vendor's full algorithm platform and how to optimally select an algorithm for each particular trade that comes in from a portfolio manager. Portware leverages artificial intelligence to help traders use execution algorithms and in some cases provides automated execution solutions that select the optimal control parameters on algorithms.
"Our work really has focused on two objectives: the first is to find an optimal execution schedule for each trade, and the second is to interact with the order flow more efficiently to avoid the harmful effects of high frequency trading (HFT)," Waelbroeck told IBTimes.
Harmful HFT
Applying machine learning to mitigate the harmful effects of HFT is dynamic process which involves controlling the parameters on execution algorithms, avoiding the orders sent to the market essentially being taken advantage of by automated trading systems.
Waelbroeck said this is done by building prediction models that predict the forward order flow in the market and then select algorithms determined to operate efficiently in the expected future order flow.
Order flow is the process by which price moves. A market participant or group of market participants execute enough market orders in sufficient size to consume the liquidity of the best bid and offer at a given and time and as a result the price moves.
Waelbroek said: "If you are buying stock, and you could make a prediction that there is likely to be more orders hitting the bid side of the spread in the next five minutes, you should be able to operate an efficient algorithm that only posts limit orders and collects the spread as it executes. Whereas, using an algorithm that crosses the spread would be wasteful since you expect order flow to be coming your way."
Waelbroeck said it is essential for the market to mix different sources of order flow; it's always been one of the functions of the market and it continues to be today with HFT.
"I think the important points to bear in mind, as far as regulations are concerned (I'm not going to walk into those waters too much), but I think it's important to make sure that institutional traders and HFT traders have access to the same order types and the same types of tools.
"We certainly regard our development of sophisticated methods to optimise the control of execution algorithms as a natural response to the emergence of HFT in enabling institutional traders to protect themselves from potential harmful effects of HFT, so they can benefit from their liquidity most effectively without suffering potential harmful consequences."
Regarding a response to HFT, Waelbroeck pointed out that Portware's modus operandi is to anticipate, not to react. "If you want to deal effectively with HFT or Alpha Profiling, you need to have a forward looking view of what's coming ahead, so you really have to have a design mandate to focus on prediction modelling over the horizon where your decisions are going to take effect, to assist the traders with optimal decision recommendations that are based on an understanding of the most likely outcomes of the decision."
Alpha Profiling
Portware's Alpha Profiling methodology focuses on trade execution with a longer time horizon, which could be days or even weeks for larger trades. In the case of optimal execution scheduling there may be a lot of knowledge about the portfolio manager's historical behaviour - does he tend to place orders to buy before a bottom, for example.
Waelbroeck said: "Is there a way to combine your information about the portfolio manager's history with the current real time market drivers to have a good classification of the trade in terms of urgency - deciding whether you execute fast or take your time and execute more slowly because you are going to find better prices later on."
In terms of implementation, a portfolio manager would send through a historical data set of every trade they've done over the last two to five years, said Waelbroeck. The data set is then enriched by recreating the market factors at the start of each trade, "so we can basically put ourselves back in the situation the trader would have been in when he received each of these orders, and through back testing methods we can then determine, ex-post, which would have been the optimal execution strategy for each order.
"From that point on what you need to do is build a classification model that can associate the optimal execution strategy to each order and use that model in the future."
Waelbroeck said there's quite a large arsenal of tool for machine learning and artificial intelligence applications, and Portware uses most of them in its platform. The primary tool I would say for Alpha Profiling, he added, would be a combination of trees and Bayesian scoring engines (a method of statistical inference in which Bayes' theorem is used to update the probability for a hypothesis as more evidence or information becomes available).
Noisy data
Experts in the field of artificial intelligence and machine learning face the challenge of dealing with "noise" in market data, which can mislead.
"Machine Learning specialists are not unfamiliar with noise – it's part of the business," noted Waelbroeck. "What's a little bit more difficult in the case of trade execution is that you have to combine the effects of your own trading on prices, with the intrinsic dynamics of the system.
"In other words if you look at a trade and you find that the price moved adversely, you might say I should have traded faster because the price moved adversely. But that's not necessarily true; you have to determine first - did it move adversely because of what I did?
"So you have to able to untangle market impact from the intrinsic price dynamic, before you can make effective decisions in optimal trade execution - that's very difficult. It's an area where we have been developing an expertise in over last eight years."
AI summer
Waelbroeck agreed there is no question we are currently living through another "AI summer". But he added that even today data that is generated by the trading process in asset management operations is largely under used.
"To be fair it is very difficult to use it because it's data that entangles the effects of market impact with all of the market variables, which are notoriously difficult to predict. So it's very difficult to extract value from that data but there has definitely been a surge in attempts to leverage that data to achieve superior performance.
"I think of it as a bit like the birth of statistical arbitrage back in the day, where people found that it was possible to exploit statistical patterns to trade for profit and develop the whole branch of the hedge fund industry on that basis.
"I think similarly if you look at institutional trade execution data, there are gold nuggets there that can be mined to achieve superior performance in the same way as statistical market data was used by stat arbitrage firms."
© Copyright IBTimes 2025. All rights reserved.