New AI can detect earthquakes sooner and reduce natural hazards
New system is 13,500 times more accurate than current earthquake detection method.
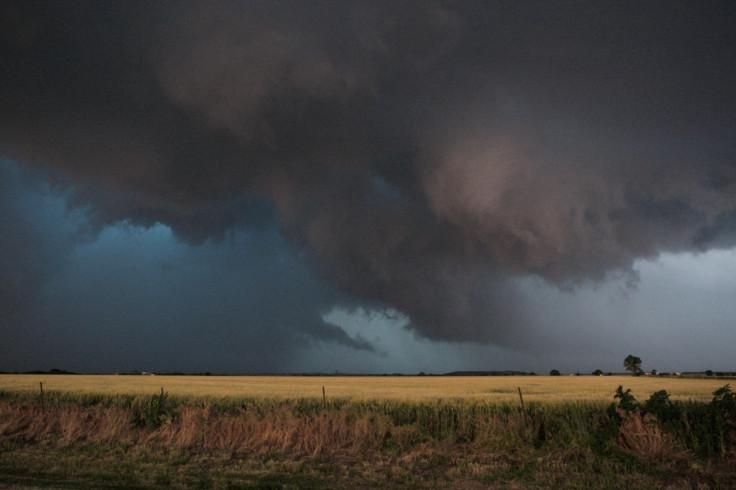
A team of researchers have built an Artificial Intelligence (AI) system that can detect earthquakes faster than any other existing devices.
Engineers from Harvard University and the Massachusetts Institute of Technology (MIT) came up with an algorithm that can predict earthquakes before they happen and reduce natural hazards. They published their findings today (14 February) in Science Advances.
Most of the existing earthquake detection methods are designed to detect medium to large earthquakes and fails to sense smaller events. That is because these minor earthquakes are buried under what is known as seismic noise. Rather than a clear noise, it defines a persistent vibration of the ground that can happen due to a number of random factors.
While seismic waves of big earthquakes are easily identifiable from seismic noise, it is trickier for lesser events.
But these smaller earthquakes come with risks too and detecting them could help reduce a number of hazards.
MIT and Harvard scientists led by Thibault Perol have come up with a system called ConvNetQuake (Convolutional neural network for earthquake detection location) that can detect big, medium and small quakes with an accuracy of 94.8%. It can also predict the location of these earthquakes with 74.5% accuracy.
How it works
In order to understand how different ConvNetQuake is from already existing models, we have to look at how traditional models work to detect big and medium earthquakes.
They are often based on waveform similarities as it enables the detection of quakes that originate from the same region and have the same source. Waveform correlation is the most accurate method available today to detect these repeating earthquakes. However, it takes a lot of time to detect long repeating series of earthquakes.
Another method, known as Fingerprint and Similarity Thresholding (FAST) does not require human supervision. It extracts fingerprints from seismic waveforms and creates a data bank of these fingerprints.
ConvNetQuake is faster than both these systems and can handle larger sets of data as well. It creates a map of probable seismic events.
Looking at how each system performed when analysing one week's worth of continuous seismic waveform data, the autocorrelation data predicted 100% of earthquakes, but took 9 days and 13 hours to do so. FAST predicts 88.1% of them in 48 minutes. It took ConvNetQuake only one minute and one second to predict 94.8% of earthquakes, as well as signposting their location.
According to the study, ConvNetQuakes is 13.500 times faster than the aucorrelation method and 48 times faster than FAST.
They tested their system in Oklahoma, where more earthquakes activity has been detected in recent years. They analysed the seismic activity of that region that occurred between August and November 2014.
ConvNetQuakes successfully predicted all 209 events catalogued by the Oklahoma Geological Survey in July 2014.
Perol's system could be extremely useful in not only detecting earthquakes but also understanding why they occur. However, it performs best when big quantities of data have already been collected, and will detect earthquakes more accurately in areas with high seismicity rates.