Which defendants are likely to be a flight risk? AI could help judges make better decisions
Algorithms can better predict what criminal defendants will do when released on bail than judges can.
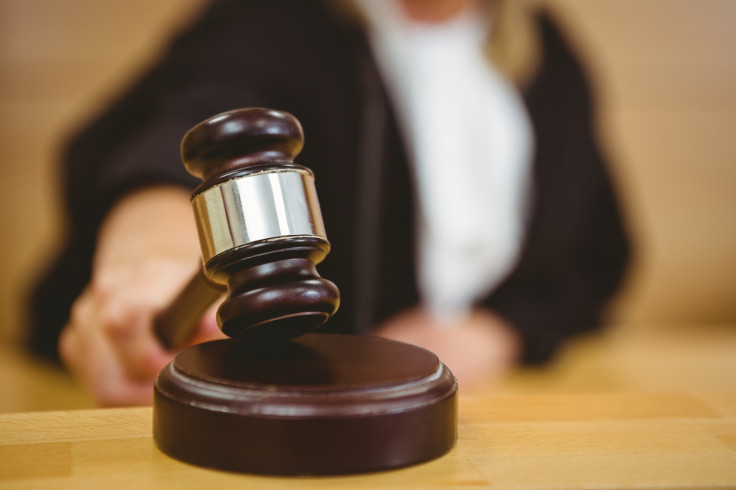
Computer scientists and economists are using machine learning to predict which criminal defendants are likely to become flight risks far better than judges can, which could help reduce crime as well as overcrowding in prisons.
Researchers from Cornell University, Harvard, Stanford, the University of Chicago and the US National Bureau of Economic Research have trained an algorithm to predict what defendants will do when they are released on bail and allowed to go home, having it look at the criminal records of hundreds of thousands of real cases in the New York City justice system.
The training dataset consisted of decisions made by judges and the outcomes of the defendants they decided to release, as well as information about each defendant, such as when and where they were arrested, what type of crime they were suspected of, how many times they had previously been convicted and what type of crimes they had been convicted of.
After the computer algorithm was trained, the computer scientists then asked it to evaluate which defendants were likely to try to run away, and when given over 100,000 cases to look at, the algorithm was able to predict which defendants were a flight risk far better than the judges had when they had made their decisions.
Since judges are human, they are unlikely to ever evaluate a defendant based on only one criteria when deciding whether they should be granted bail, since certain judges might have a specific focus on violent crimes or racial inequities.
So the researchers used econometric strategies to randomly assign cases, as a way of getting the algorithm to not just predict the best outcome, but also to see if it could predict how the judges would rule.
The results of their study show that if judges in New York City were to use software containing this computer algorithm when deciding whether to grant bail, crime could be reduced by up to 24.8% without changing the numbers of people waiting in jail. It would also be possible to reduce the number of people waiting in prison for their trial dates by over 40%, while leaving the crime rate by defendants unchanged.
The algorithm was also tested on similar data from 40 large urban counties in the US and it produced similar results to the analysis of cases in New York City.
While these results sounds like they're just meeting the status quo, the point is that the software would be really useful to highlight to judges when they might be making a really bad decision, since an analysis of the judges' performance so far revealed that some defendants released did go on to commit another crime or fail to show up in court.
"By focusing the algorithm on predicting judges' decisions, rather than defendant behaviour, we gain some insight into decision-making: a key problem appears to be that judges to respond to 'noise' as if it were signal," the researchers concluded in their paper.
"These results suggest that while machine learning can be valuable, realising this value requires integrating these tools into an economic framework: being clear about the link between predictions and decisions; specifying the scope of payoff functions; and constructing unbiased decision counterfactuals."
© Copyright IBTimes 2024. All rights reserved.