G4S, Lloyds, Burberry: How artificial intelligence spots insider transactions before a stock hits freefall
G4S, Lloyds, Burberry: How artificial intelligence spots insider transactions before a stock hits freefall
Machine learning algorithms can warn investors when a particular stock is going to fall by predicting likely instances of insider transactions (when information that's not in the public domain is capitalised upon by people in the know). This can be done by analysing previous occasions when company insiders did apparently well-timed trades in their own stocks, and recognising these patterns.
This might seem deceptively simple, but it isn't, explains Tom Doris, CEO of OTAS Technologies, a London-based market analytics and machine learning trading system. While company executives are required to file details of transactions in their company's stock, most insider trades are few and far between: a needle in a haystack.
Doris told IBTimes UK: "We look at all of the insiders, the directors of companies, and we see all of their historical transactions in their own stock. If you are the chief financial officer of Vodafone, any time you buy or sell Vodafone stock, you're obliged under regulation to file details of those transactions. So that would include the amount that you bought or sold, when you did it and what price you got and the reason, if any, for the transaction. There is an enormous database of all of these transactions for all of the world's listed stocks and we go and we basically back-test all of the insiders and we find the ones that are apparently good at timing their own stocks."
G4S at the Olympics
A good example of insider trading activity detected by OTAS prior to a big price fall was when insiders at security firm G4S all started selling their stock right before the Olympics announcement that was very damaging to the company's share price – detectable in advance because of historical precedents.
"Normal back-testing techniques wouldn't really have uncovered those kinds of signals," noted Doris. "But the new techniques that we apply now can take a very small number of data points and identify when there's some suspicious activity going on or some people seem to be trading off material inside information that's not yet public. So the next time this person buys or sells stock, we can say, 'hey Mr portfolio manager, this guy at G4S, or at Tesco or at Pandora, or any of these other companies – every time he sold stock in the last five years it's been very bad for the stock, therefore you should be paying attention to this transaction.'
"It's deceptively simple in some ways. But the problem is that an insider typically will not make a lot of trades so if you use old style statistical techniques on a guy who has had two or three trades in his lifetime, it will just come back as inconclusive, even if he has gotten all three of them right. It's not enough of a data set to say conclusively that he's trading off inside information. Whereas, if you or I look at the price charts and see that the three times over the course of five years that he has traded the stock, it has jumped up significantly within a month of him trading and he caught three out of the five major jumps in the stock over that five year period. You and I know immediately that it looks suspicious and it looks like he got information.
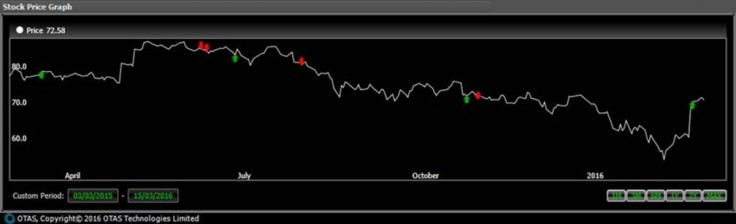
"So a lot of it is taking things that humans are quite good at and translating that back into a new statistical methodology and using new techniques to kind of embed that domain expertise that comes very naturally to us on a single basis. When you systematise it and put it into an algorithm obviously you can run it on thousands of stocks and hundreds of thousands of transactions."
OTAS sees opportunity in applying machine learning techniques to filter, highlight and push concrete evidence to human decision makers where there are big gaps between a historical situation; where it would not be feasible for a portfolio manager to stay on top of all of the news and all the research for a given company from 20 years ago. Doris said this is an area not really policed in any way; a lot of companies have lock up periods ahead of an earnings announcement where there is material non-public information and they prevent their employees from trading their stock. "In terms of systematic ways to identify people who appear to have an uncanny ability to get their trade timing right, we are not aware of any other system in existence that does that other than the one that we have invented."
High Frequency Trading
Doris, who has worked for hedge funds and holds a PhD in neural networks, points to misconceptions about how AI and machine learning is used to assist people at hedge funds, or quants in general, to predict future price moves. This is partially the case on a very short time scale, but actually the people who make the most money out of high frequency trading, for instance, are more interested in predicting if somebody is going to buy stock aggressively in the next couple of seconds. "The idea of predicting what the price is going to be, or predicting what the next three months will unfold, is not really something that anybody in finance really spends a lot of time on. The ones who are very good at quant and high frequency can have predictions that go out for a couple of seconds or a few minutes at the most.
"The markets are reasonably efficient and are very good at pricing in information and HF traders have a role to play in that. It's a very good way of getting information about a stock into the public domain; that's how they function and as a result material information doesn't really stay private for the length of time that it would need to stay private in order to have a prediction that would last for anything more than several minutes. It's by virtue of the markets actually functioning correctly that those opportunities simply don't exist."
He also pointed out that global investment houses with billions under management have strict investment mandates which must be adhered to. They tend to be more interested in flagging risk and volatility and avoiding the torpedoes in their portfolio, than some brand new black box signal guaranteed to make money. "Somebody running a portfolio at a big fund manager with tens of billions of dollars in equities might have 100 to 200 positions in stocks and two or three of those positions in any given year are going to be down 25%, and they are the ones that really hurt the performance. The other 100, they are going to track the market more or less and you might have a little bit of skill in terms of loading up on some of the better ones, but in the long run it's limited. It's all about trimming the losers. So if you have better systems that can identify when there is a risk of those kind of draw downs, that's really where the juice is and the value added."
Hedge funds and AI PhDs
There has been plenty of ink recently about hedge funds secretly incorporating AI divisions. Doris takes a philosophical view: "I think there has always been more hype than action around this stuff. It's a great way of building your assets and asset allocators are possibly the root cause of this; they are prone to being disproportionately impressed by a roomful of PhDs claiming that they are doing ground-breaking work.
"It's something that allocators look for and therefore the hedge funds respond to that – whether they are doing it or not, they will say they are. And usually you can find some area of your operation which is doing something that can be characterised as AI or ML and they roll those out whenever the allocators or the investors come to town. But these days you probably need a room of PhDs just to stay on top of your risk and compliance requirements, never mind managing portfolios."
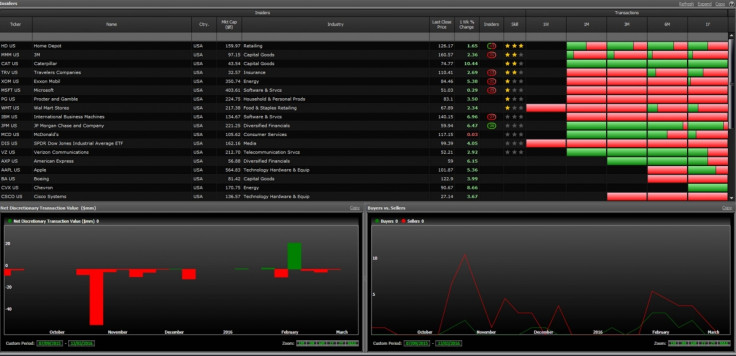
© Copyright IBTimes 2025. All rights reserved.