Next generation of machine learning rockstars will trade Google and Facebook for top secret hedge funds
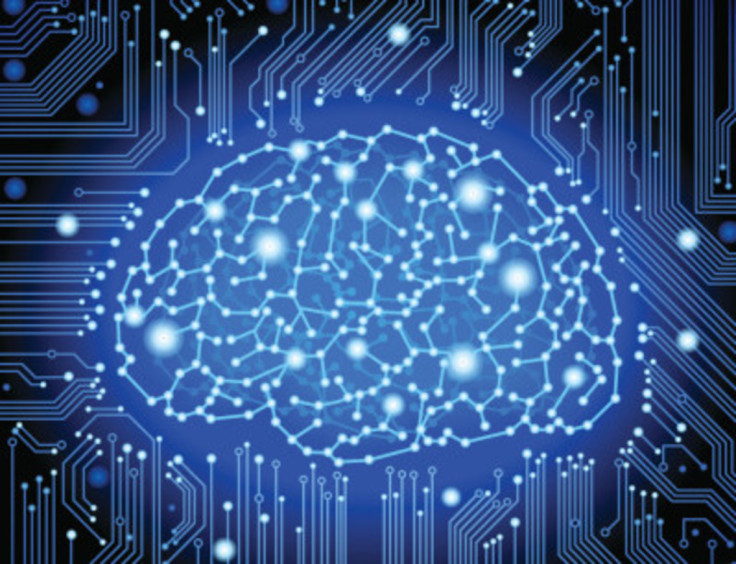
We are on the cusp of an exponential shift in machine learning, the ability of a computer to automatically refine its methods and improve its results as it receives more data. For the last couple of years, technology giants such as Google, IBM and Microsoft have been in an arms race to construct artificial neural networks that mimic the human brain. Increased computing power, combined with access to very large data sets and advancements in machine learning algorithms, have augured in this new era. We can expect to see a surge in deep learning startups focused on areas such as speech and object recognition, robotics and finance.
In the secretive world of hedge funds a very large artificial intelligence play involved Bridgewater Associates, the world's largest hedge fund, which launched a six-strong AI unit led by David Ferrucci, who joined the fund at the end of 2012. Ferrucci led the IBM team of engineers that developed Watson, the computer that beat humans on Jeopardy, the US television quiz. (Incidentally, now that Watson has won Jeopardy, it has enrolled at medical school: Dr Watson will be able to sift through more data and make faster diagnoses than an army of human doctors).
Geoffrey Hinton
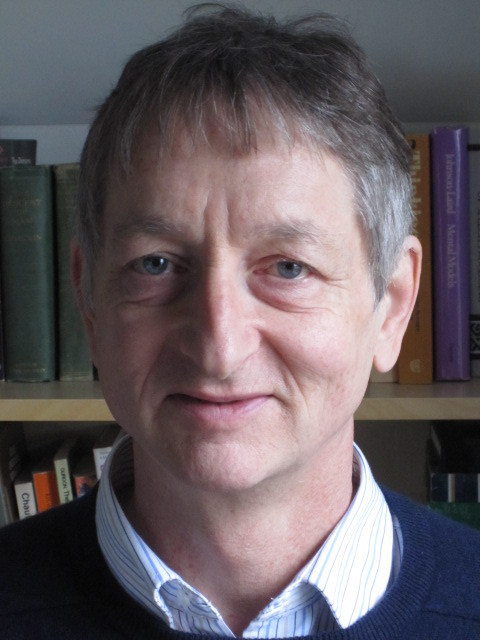
British-born Geoffrey Hinton is known as the godfather of neural networks. He spends several months each year working full-time for Google in Mountain View, California as a Distinguished Researcher. The rest of the time he can be found at the University of Toronto, where he is a Distinguished Emeritus Professor. Hinton is a cognitive psychologist and computer scientist noted for his work on artificial neural networks. He is a central figure in the deep learning movement and a prolific researcher: his CV, which lists all of his publications, is 41 pages in length. Interestingly, he is also the great-great-grandson of the logician George Boole, whose work forms the foundation of contemporary computer science.
Bridgewater, which has $165bn (£118bn, €150bn) under management, is set to create trading algorithms that make predictions based on historical data and statistical probabilities, according to some investigative reporting from Bloomberg. Hedge funds typically don't want to talk about what they are up to, and their forays into machine learning are no exception.
Guillaume Vidal, CEO of Walnut Algorithms, which applies machine learning to financial markets, told IBTimes UK: "It's hugely opaque. People really don't want to give away anything. In the financial sector, you will find very few people talking."
The likes of Geoffrey Hinton or Demis Hassabis at Google can do interviews and roundtables and are allowed to publish their research, but you won't hear about what's happening within the hedge funds, said Vidal.
A hedge fund well known for having a history of developing learning algorithms is New York-based Renaissance Technologies. The fund's co-CEOs Bob Mercer and Peter Brown developed language-recognition programmes at IBM before they joined Renaissance. Another example is Two Sigma; one of its co-founders, David Siegel, has a PhD in computer science from Massachusetts Institute of Technology's AI laboratory.
Demis Hassabis
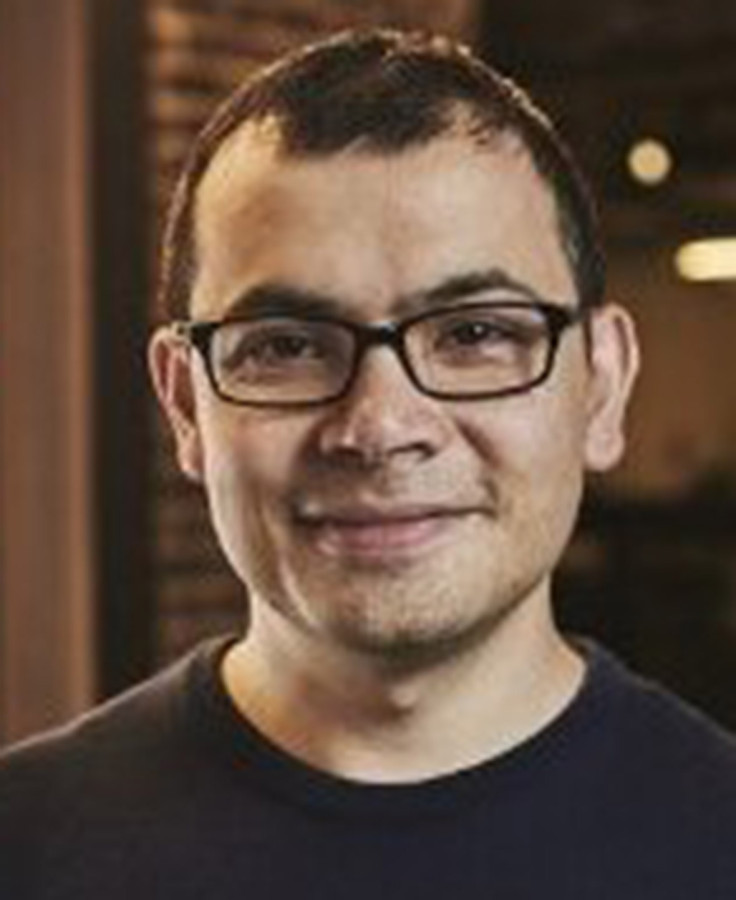
Greek-Cypriot Demis Hassabis, who grew up in north London, is a former junior chess champion and games designer. He co-founded DeepMind Technologies while at University College London, which specialised in building general-purpose learning algorithms. In January 2014, DeepMind was acquired by Google for £400m. Hassabis is now vice-president of AI projects at Google. In October 2015, DeepMind, with the AlphaGo programme, achieved an AI breakthrough by beating the European Go champion.
Other notables include BlackRock, which announced in October 2015 that it was in talks with Google to facilitate AI-based research. The fund manager called it "a very exciting joint-venture". Earlier in 2015, BlackRock hired Bill MacCartney, a former Google scientist, to help it apply machine learning to quantitative-investment strategies.
Another big play is JPMorgan Chase & Co's hedge-fund business Highbridge Capital Management ($24bn AUM). The fund is known to be working with San Francisco-based Sentient Technologies to develop investing strategies using AI. Financial firms seem to be turning to external partners to conduct this sort of research, while also scrabbling to recruit AI scientists and stay up to date in terms of the revolution in data science and machine learning.
Vidal added that the barriers to young firms employing this type of technology have come down. The complexity of the algorithms has evolved, and computers that might have cost $1m 15 years ago can be bought for a few thousand dollars today.
Andrew Ng
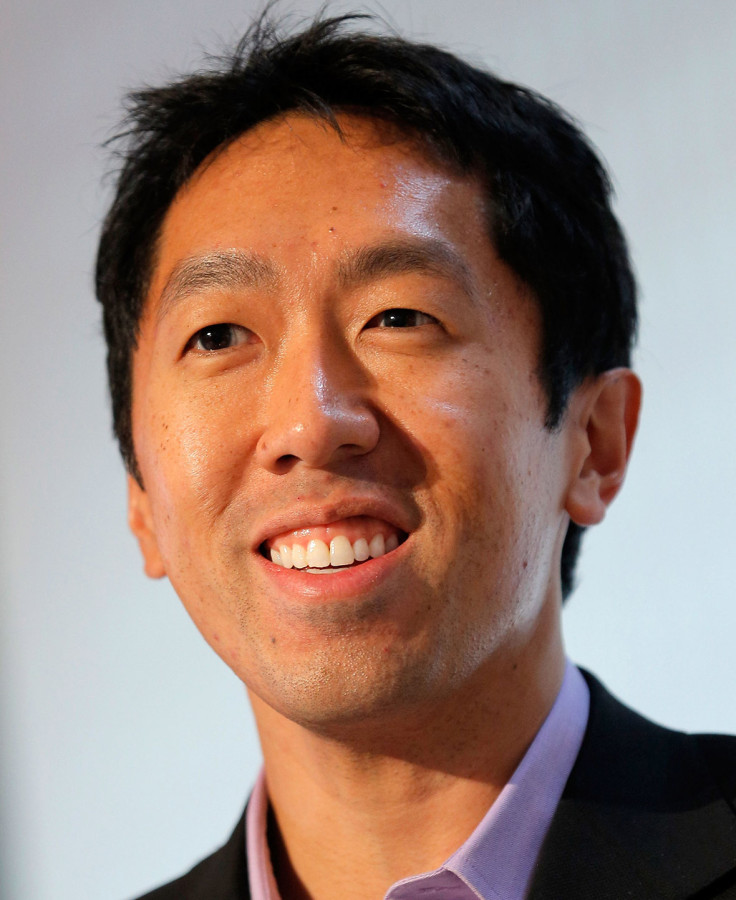
Andrew Ng, an associate professor in the department of computer science at Stanford, founded the Google Brain project at Google in 2011. It used Google's distributed computer infrastructure to develop very large scale artificial neural networks, which learned to recognise high level concepts such as cats, after watching just YouTube videos; it was never told what a cat is. Ng left Google in May 2014 to become chief scientist at Baidu, sometimes referred to as the 'Chinese Google'. He works out of the Silicon Valley-based Baidu Research.
He said: "The skills to be able to code, or to use, an algo that has already been tested and exists is not that difficult. You just need to have a little bit of statistics and maths background; if you know how to code and you use code libraries that already contain the algos that you just need to parameterise. These are people we call data scientists – the people who use algos to do data exploration."
He said the difficult part is getting mathematicians that understand the algorithms well enough to be able to do some form of research to apply them to finance. "We at Walnut need quant finance skills as they are different from other skills. We need coding skills as that is the infrastructure, to get the data, clean it, put it in data frames. Data frames are pre-processed into the machine learning which outputs information, and that is processed to create trend strategies from it."
The combination of IT infrastructure, machine learning, mathematics and quantitative finance skills make it difficult for someone to just come up with a machine learning system all of a sudden; it takes some time, said Vidal.
Yann LeCun
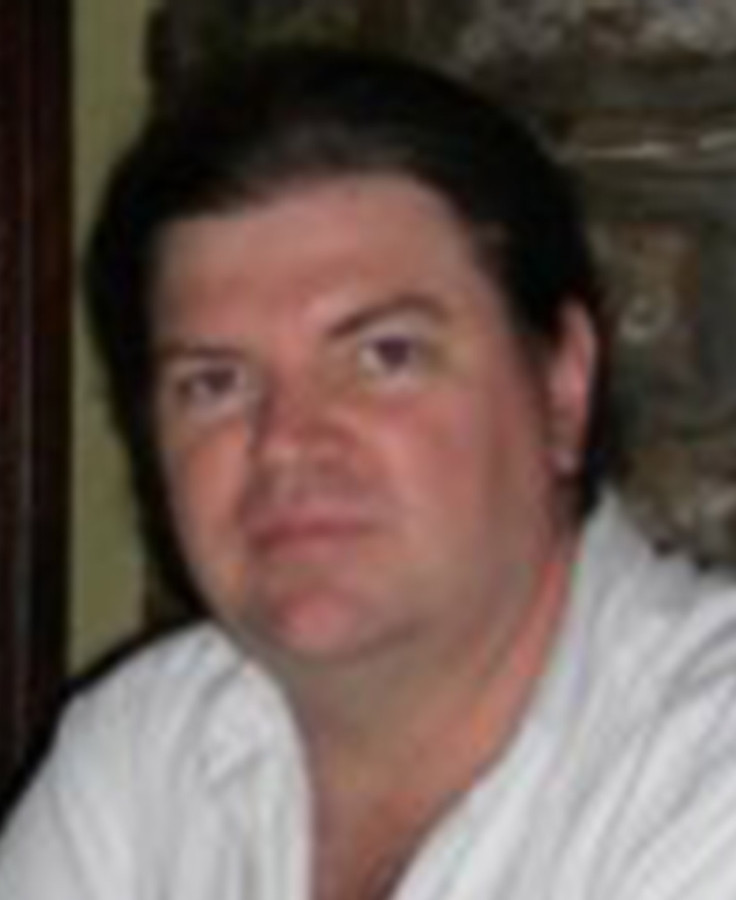
Yann LeCun, a scientist who has contributed to machine learning, computer vision and mobile robotics, became the first director of Facebook AI Research in New York City in December 2013. He was the also the founding director of the NYU Centre for Data Science, a position he stepped down from in early 2014. He co-developed the Lush programming language with Leon Bottou.
Typically, machine learning trading strategies in finance look at past performance. A straightforward trading algorithm might take the best performing 20% of stocks from the S&P 500 from the previous week and short them, and the bottom 20% and go long on them. However, big-data analytics can have endless possibilities, beyond fundamentals and macro-economics into behavioural data, weather systems and so on. "Some quants even start looking at weather patterns effecting crops, for example. This is all quantifiable. You can look at satellite pictures of crops or even supermarket parking lots to verify if a lot of people are actually going to supermarkets. All of this data can actually be digested, explored and made sense of by machine learning algorithms," said Vidal.
Yoshua Bengio
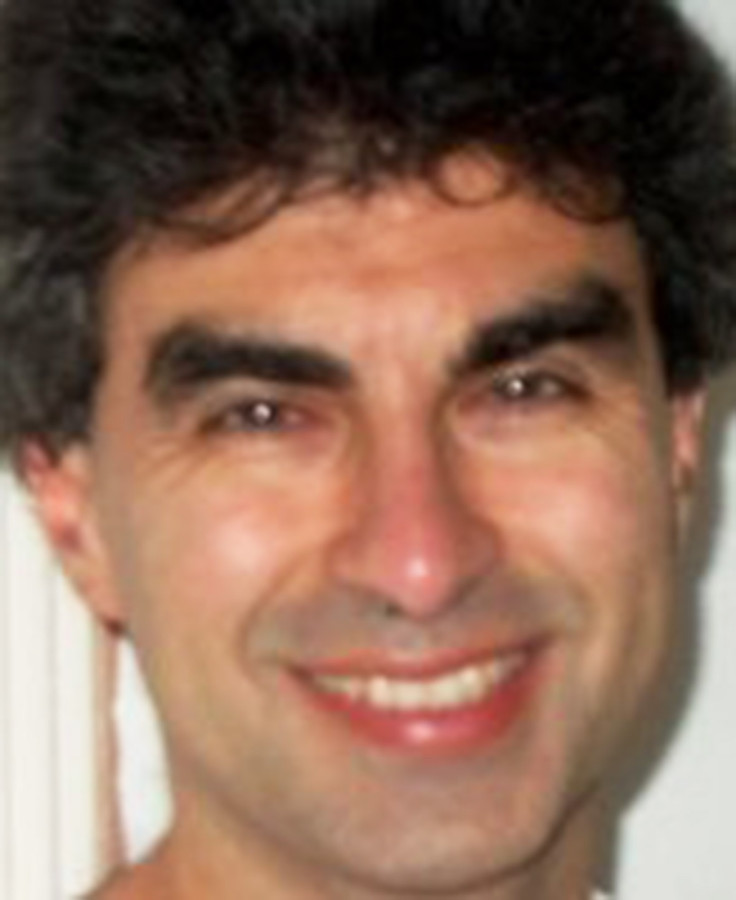
French-born Canadian Yoshua Bengio is a professor in the department of computer science at Université de Montréal and holds the Canada Research Chair in Statistical Learning Algorithms. Bengio is another notable in deep learning. He was a post-doctoral fellow at MIT and AT&T Bell Labs.
Referring to the established rock stars of machine learning – Geoffrey Hinton, Demis Hassabis, Yoshua Bengio, Yann LeCun and Andrew Ng – Vidal said: "Today when there's a conference involving one of these superstars of artificial intelligence there are going to be a lot of people going. And it's really recent; these names were not really known outside of academia two or three years ago."
However, not everyone agrees that the men mentioned here deserve to be thought of as godfathers of machine learning.
Juergen Schmidhuber, Scientific Director of the Swiss AI Lab IDSIA and Professor of Artificial Intelligence, Univ. Lugano, Switzerland, told IBTimes: "This is really an insult of the true pioneers of the field. I critisised the self-proclaimed "Deep Learning Conspiracy" of Hinton, Bengio and LeCun in this now pretty famous review. This priority dispute is well-known among experts in the field, but apparently not yet among journalists."
© Copyright IBTimes 2025. All rights reserved.