Why Thomson Reuters' introduction of enterprise graph analytics to its global customers is a big deal for big data
Older technology hasn't been able to keep up with data volumes and demand for insights
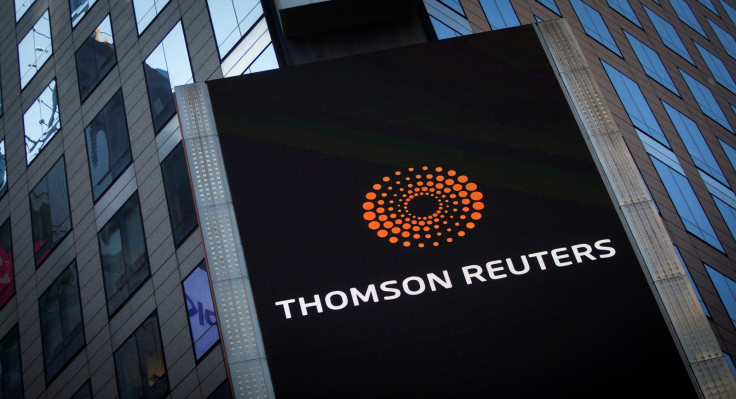
The announcement by financial information provider Thomson Reuters this past week that it will offer a "Knowledge Graph feed" to its customers is big news for big data.
Why? For the first time a highly influential data provider has publicly acknowledged the effectiveness of graph-based information – as opposed to traditional database tables – in finding answers, insights and value from incredibly vast amounts of connected data.
While many in the financial services industry and other sectors have been using graph-based technology in their operations for several years, no one has touted the technology as part of a marketing strategy to attract new customers and keep existing customers happy – until now. The latest Thomson Reuters offering demonstrates that this new way of presenting and understanding data has gone mainstream.
The evolution from tables and rows to graph-based queries is a sign that traditional technology is not helping people understand today's ever-growing stores of data, and is likely the driving factor behind Thomson Reuters' decision to make the move.
For those of us who are not data scientists, knowledge graphs are interconnected or linked representations of data described semantically, i.e. with context and human meaning, that can be mined in a much more intuitive way.
If you think about it, most of us discover answers through associations and connections. For example, let's imagine that you were asked, "What was the best meal you ever had in your life?" You might first think "swordfish," which links to Maxim's restaurant in Paris, which links to New Year's Eve, and so on to form the memory.
We consciously or otherwise ask ourselves follow-up questions to flesh out memory: What was the wine? What were we wearing? What was the conversation?, etc. Every related fact deepens the picture and leads to other trains of thought.
Google, Facebook, LinkedIn and others have leveraged this predilection of the human mind to connect people, places and events in their software, interweaving personal relationships and interests with just about everything else in the world. Although it may not appear that way on the surface, these online giants are representing data as a graph or network of interconnected information. Now with recent improvements to data processing power, this extraordinary search capability is available within your company to solve business problems.
Making the news even better is that the Thomson Reuters Knowledge Graph is based on open standards initiated by Tim Berners Lee, inventor of the World Wide Web. Open standards make it much easier to share data and create a human-friendly way to understand that data.
According to Thomson Reuters, the Knowledge Graph uses "linked-data principles of the Semantic Web...in accordance with the Resource Description Framework (RDF)." This is especially important as the Financial Industry Business Ontology (FIBO) standards defining graph-based business meaning are also being adopted. Similar data standards used to share a business level understanding of data in other industries, such as CDISC for drug research and HL7 FHIR in healthcare, are being adopted as well.
Standards that share the meaning of data also enable business users without query language skills to develop sophisticated interactive graph-based searches and analytics in real-time across very large, interconnected data sets. Users will start to ask previously unasked questions that help them find the "unknown unknowns," to uncover valuable insights they didn't even know existed.
Other companies and industries will take note of what Thomson Reuters is doing and discover the value of using enterprise knowledge graphs for themselves. The wider business market will now know that viewing large volumes of connected data in graph form comprising hundreds of billions and trillions of facts is now possible.
In recent years Cambridge Semantics has seen broader adoption of this technology in the financial industry, dealing with problems such as insider trading and regulatory compliance or achieving a deeper understanding of their customers, as well as in pharma research for accelerating drug development. An array of other data-intensive industries is exploring graph-based discovery and analytics as well, including manufacturing, oil and gas, and retail.
Graph-based data analytics is the next evolutionary step for big data as companies move toward something called "enterprise data fabrics." The enterprise data fabric will make all the data in a business instantly available to any authorised user with the ability to quickly organise and understand what the data means.
Historically, most enterprises leverage less than 20% of their data because of the considerable amount of this volume is only available as text which is hard to combine with structured sources. Graph technology is key to unlocking and combing the remaining 80% of unstructured textual data. We are entering an age where business will have access to all the data they want, whenever they need it.
There's a reason why data is often called the new gold these days. And Thomson Reuters has just set its customers on a path to collect it.
Sean Martin is Chief Technology Officer of Cambridge Semantics.
Thomson Reuters will be talking about big data at Newsweek's AI and Data Science in Capital Markets conference on December 6-7 in New York, the most important gathering of experts in Artificial Intelligence and Machine Learning in trading. Join us for two days of talks, workshops and networking sessions with key industry players.