New Research Highlights the Realities of AI Adoption in Business
Mesh-AI's new research report, 'The State of AI in the Enterprise', highlights the realities and challenges of AI adoption faced by businesses.
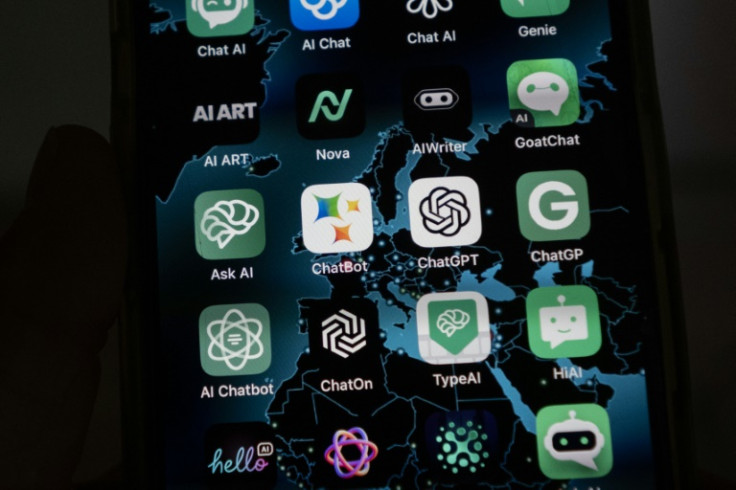
In today's world, advances in artificial intelligence (AI) present both opportunities and challenges for businesses seeking to adapt to new technological capabilities.
In an effort to establish the facts, a new research report by enterprise consultancy Mesh-AI presents findings from the responses of over 200 business leaders which shed light on the realities of AI adoption. In order to delve deeper into the findings of their research, I spoke to Ben Saunders, Mesh-AI's Chief Customer Officer.
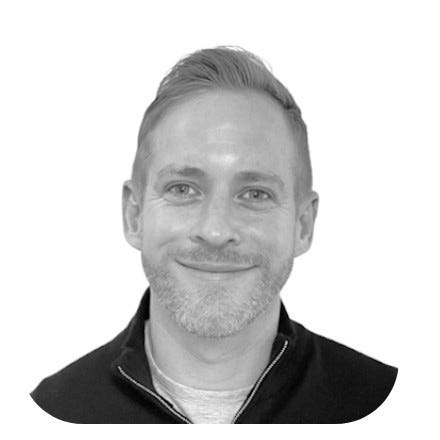
According to Saunders, with the "amplified curiosity" created by the development of generative AI solutions like Chat GPT, "there are a number of organisations that are trying to get onto the AI bandwagon". However, despite the excitement created by new forms of technological innovation, businesses don't necessarily possess an understanding of "how that technology can be applied to solve business problems".
Accordingly, Mesh-AI's report reveals that a lack of understanding of technology is the most cited obstacle to AI adoption, with 22 per cent of respondents ranking it as the number one barrier. Other barriers include regulation and compliance, competing priorities for businesses, lack of funding, lack of strategy, lack of technical skills and lack of executive sponsorship.
AI Strategy and beyond
Mesh-AI's report reveals that 90 per cent of enterprises believe that AI is moderately, very, or extremely important for their business model. However, despite this, not all of Mesh-AI's respondents reported that they possess a clearly defined and well-understood AI strategy.
Only 15 per cent reported they possess a strongly defined and well-understood strategy. In contrast, 18 per cent said they had no clear strategy at all. Furthermore, 35 per cent of respondents reported they possess a somewhat defined and well-understood AI strategy, whilst 32 per cent reported that they possess a poorly understood AI strategy.
To understand more about the factors businesses need to consider in order to effectively AI solutions, I asked Saunders to explain what constitutes an effective AI strategy. He made several key points.
Firstly, organisations need to consider the relationship between their business strategy, data strategy and AI strategy.
Saunders explained: "It is really important to ensure that all of those three things are symbiotic and complementary in terms of the impact that they are looking to add to customers and the value they are looking to create for the business."
Secondly, it is important to create an "AI culture within the business", which is grounded in AI "literacy and awareness". Moreover, that means creating "a clear understanding and appreciation of your organisation's desire to apply AI, where you want to apply it, and most importantly, where you don't want to apply it", Saunders illustrated.
Thirdly, "an AI strategy has to be founded on strong ethics and cultural values" that fit alongside "the principles and beliefs of the business". This is important for ensuring that there is not a "seismic misstep" in the application and use of AI. In other words, that businesses achieve what they intend to in terms of their business objectives, rather than causing unintended disruption and negative consequences.
Fourthly, following from the above, one key factor is data. Organisations intent on using AI need to consider how they are going to "democratise data access" and "increase the trust and integrity" of their data, Saunders explained.
This is important to ensure that when "more sophisticated" machine learning and AI capabilities are adopted, organisations have the capacity to "validate the origin and the locality of where certain data sets have come from". This means developing an "end-to-end capability, whereby you can have full traceability around the who, the what, the why, the when and the how".
According to Saunders, based on what Mesh-AI have seen in enterprise organisations over the past years, "there is a significant distrust in data". In many enterprise organisations, it is not always the case that data is handled in an "optimal manner".
Moreover, not all organisations ensure that data is "stored in systems of record" through which data quality can be ensured using "automated testing frameworks", and where "the accuracy, the freshness and the completeness of the data" can be guaranteed.
Where businesses fail to get their data in order, the risk is that employees start to rely on personal copies of data, with "dark data starting to manifest itself outside of the core hubs" of organisations, Saunders explained.
Crucially, when organisations do not have their data stored in an "accessible democratised architecture", they are prohibiting themselves from applying machine learning and artificial intelligence "at scale". Moreover, this underscores the importance of educating business leaders about "getting data in order" in addition to ensuring that their AI strategy and the data strategy are complimentary.
Maturity and familiarity
In addition to providing insights into AI strategy, Mesh-AI's report also reveals the extent of AI maturity and familiarity amongst different businesses. Interestingly, the report indicates that some industries are more advanced than others when it comes to their understanding and adoption of AI.
Naturally, computer and technology firms expressed the highest levels of AI maturity, with 18 per cent of firms rating their maturity five out of five. The report also indicates that the financial services sector possesses significant levels of AI maturity. Whilst only three per cent of financial services firms rated their AI maturity five out of five, the majority of respondents rated their organisations three or four out of five.
Considering the financial services industry, Saunders illustrated that "you would probably say that historically that is one of the most mature and high performing in respect of AI adoption". This can be put down to a history of using "quantitative analytics" as well as "requirements around risk management" relating to "the veracity and the velocity of the financial markets".
However, the responses of firms in the energy and utilities industry indicated a lesser level of AI maturity. A notable 30 per cent rated their AI maturity one out of five, with just nineteen per cent rating their maturity four out of five.
However, in terms of "AI readiness", the energy and utilities industries have been "on the laggards", according to Saunders. The "race to net zero and the energy transition that the world is going through" means there is a clear motivation to take advantage of AI capabilities.
Talent and skills
The challenge of tapping into the opportunities that AI brings is testing the skills and talent of enterprises. Right now, "there is a significant dearth of talent at this time across all sectors", Saunders illustrated. For example, in the UK space sector, AI and machine learning skills are particularly in need. Moreover, Mesh-AI's report refers to the historical challenges businesses have faced in accessing the right skills.
However, the report also reveals that businesses have some degree of confidence in their in-house skills when it comes to safe and secure AI innovation. Their results are fairly evenly distributed from low to high confidence.
Specifically, 39 per cent of enterprises stated that they were somewhat confident, 26 per cent stated they were mostly confident and a further 19 per cent stated they were slightly confident. However, just seven per cent expressed extreme confidence and nine per cent reported they were not confident at all.
Moreover, effective AI adoption requires different skills within enterprises. Firstly, as alluded to in the points about effective AI strategy, a key starting point is accessing the vision that can allow a business to understand where it could start to use AI. That is, both to improve the way it operates internally and what it offers to customers externally. In other words, a business needs the necessary experience, skills and awareness to "re-imagine" how it could operate. This constitutes "a first skill" in itself, Saunders explained.
Naturally, any process of organisational change requires the ability to challenge the status quo. This is no less true when it comes to AI adoption which demands the ability to find alternative ways of operating, compared to how things have been done traditionally.
Therefore, according to Saunders, it is important to involve individuals with a capacity for curiosity and "that can be challenging in a positive way". This is important for investigating "how business processes can be re-augmented through the deployment of AI", a capability that constitutes "a skill in itself".
Once a business has an understanding of how AI could be applied, the task is then one of "building your AI-enabled software end to end". This constitutes "another unique skill", described Saunders.
What are the risks?
One key question is how organisations in critical national industries (for example, in energy and utilities or financial services), can safely adopt AI capabilities without putting themselves, consumers, and the wider economy at risk.
Firstly, as explained above, businesses intent on using AI need to ensure they have built sufficient "muscle memory" to be able to understand where AI can be effectively and safely applied. Muscle memory means "the skills and experience of your organisation" that enable it to understand AI. For example, where it should and should not be applied, explained the Chief Customer Officer of Mesh-AI.
Secondly, businesses need to ensure they have "the technology capabilities in place" that provide "full end-to-end visibility" in their use of AI. For example, who is involved, what specific AI capabilities are used, why they are used, when, and how.
Ultimately, according to Saunders, AI needs to be both "explainable" and "traceable" so that "should regulators come knocking", businesses are able to provide "an almost an on-demand auditable capability".
Once businesses have built sufficient "muscle memory" to be in a position to think about using AI, they can first "look internally" to understand where AI can be applied, "prior to looking at ways it can be applied to customer-facing services". Doing this allows a business to "build a better understanding" of its "control mechanisms". Moreover, it makes sense to first apply AI in low-risk scenarios and consolidate skills and experience over time before applying AI in more challenging and risk-orientated ways.
Regulation
Inevitably, a key factor for AI risk management is the role of regulation and best practices. Regulatory scrutiny and established best practices have a clear role to play in externally guiding organisations intent on adopting AI capabilities.
Regulation and compliance are sighted by Mesh-AI's report as the second biggest barrier to the adoption and deployment of AI in business, with 15 per cent of respondents expressing concern. However, the report also explains that most businesses deem regulatory scrutiny only moderately impactful on their plans (42%). Only four per cent viewed regulatory scrutiny as significantly impactful, with a further 17 per cent viewing it as substantially impactful.
According to Saunders, there has been demand amongst "a number of organisations" for regulators to produce guidance on what constitutes "an unacceptable use of AI". Crucially, AI regulations are still in the process of evolving.
Mesh-AI's Chief Customer Officer referred to the EU AI Act as an emerging form of AI regulation that "is giving organisations guidance around how AI can be applied". However, proposed back in April 2021, the EU AI Act is yet to become law.
Lastly, despite the evolving nature of AI regulation, it is important to acknowledge that when it comes to machine learning, "there are a set of best practices that have been somewhat well established for a number of years".
Saunders referred to the NIST and ISO (International Organization for Standardization) frameworks as two examples "which many organisations should consider following if they are looking towards the adoption of machine learning."
© Copyright IBTimes 2025. All rights reserved.