How big data and exascale supercomputers could crack cancer's code
Next-generation computing power and machine learning could lead to promising treatment breakthrough.
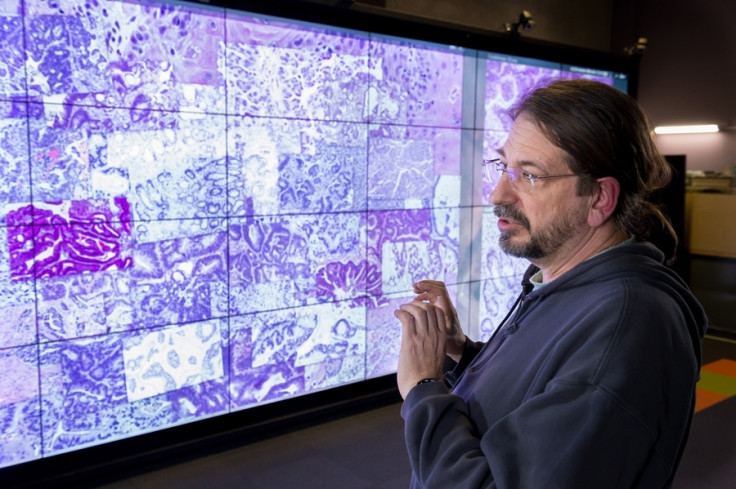
The amount of data doctors need to handle during cancer diagnosis and treatment is so vast that regular computers are struggling to cope. To help crack the complicated process doctors are turning to exascale supercomputers, which can perform up to a quintillion calculations per second, and machine learning that could one day lead to a breakthrough to beating cancer.
More than eight million measurements are taken from the biopsy of a single tumour in a typical cancer study as doctors analyse how the cancer is behaving, how it is responding to drug treatment and how the patient's body is being affected. The data is overwhelming.
The Argonne National Laboratory, a research lab out of the University of Chicago, has identified the rising promise of computational power from exascale systems being developed which could help provide 'precision medicine'.
"Precision medicine is the ability to fine tune a treatment for each patient based on specific variations, whether it's their genetics, their environment or their history. To do that in cancer, demands large amounts of data, not only from the patient, but the tumour, as well, because cancer changes the genetics of the tissue that it surrounds," said Rick Stevens, Associate Laboratory Director for Computing, Environment and Life Sciences for the US Department of Energy's (DOE) Argonne National Laboratory.
Combining human medical research and computing power the researchers are working on deep neural network code CANDLE (CANcer Distributed Learning Environment) to help prognosis and develop a treatment plan specific to an individual patient.
Currently, for treatment strategy cancer researchers have to manually sift through stacks of data interpreting medical records and clinical reports, then keep on top of the cancer's development using databases maintained by small teams.
CANDLE's machine learning could compile and compute all the known data ever created on cancer functions and drug reactions within certain individuals to identify what would be the best treatment plan. It will aid researchers in understanding the molecular basis of key protein interactions, develop predictive models for drug response and offer optimal treatment strategies.
"We are trying to devise a means of automating the search through machine learning so that you'd start with an initial model and then automatically find models that perform better than the initial one. We then could repeat this process for each individual patient," said Stevens.
To do all this, though, an exascale computer would be needed as it requires the highest-performance available. Argonne believe exascale systems could be able to run its CANDLE application 50 to 100 times faster than today's most powerful supercomputers.
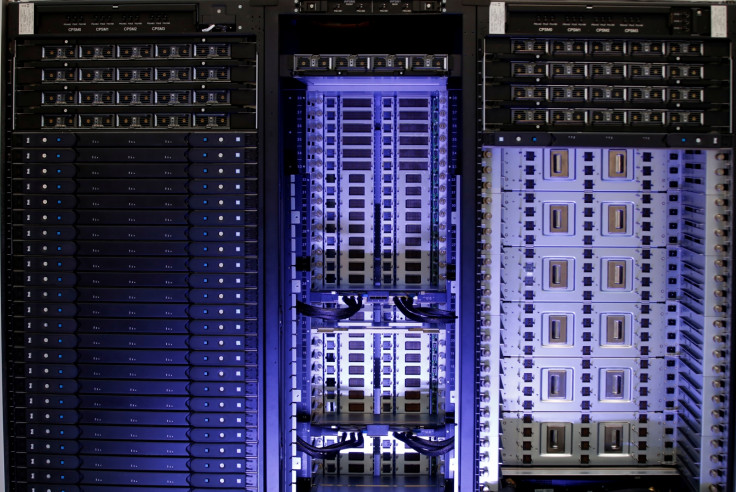
"The types of things researchers would like to accomplish now require a lot more data, capacity and computing power than we have. That's why there is this effort to build a whole new framework, one focused more on data," said Paul Messina, director of ECP.
"CANDLE will play an essential role in the development of applications that drive this framework, creating the ability to analyse hundreds of millions of items of data to come up with individual cancer treatments."
The promise is an exciting development, however it will be a number of years until exascale computers will be widely available. Currently, China owns the only exascale computer after announcing the world's first exascale prototype in January 2017. A fully functioning model of the Tianhe-3 won't be ready for another couple of years.
It is understood the US is also in the race to develop an exascale supercomputer with the government working with AMD, IBM, Intel and Nvidia to get it operational before China or anybody else. However, the timeline for this is expected to be at least 2021.
© Copyright IBTimes 2025. All rights reserved.