Homer Simpson defeats Google's all-powerful DeepMind artificial intelligence
Super computer not smart enough to visually recognise many of Homer's signature actions.
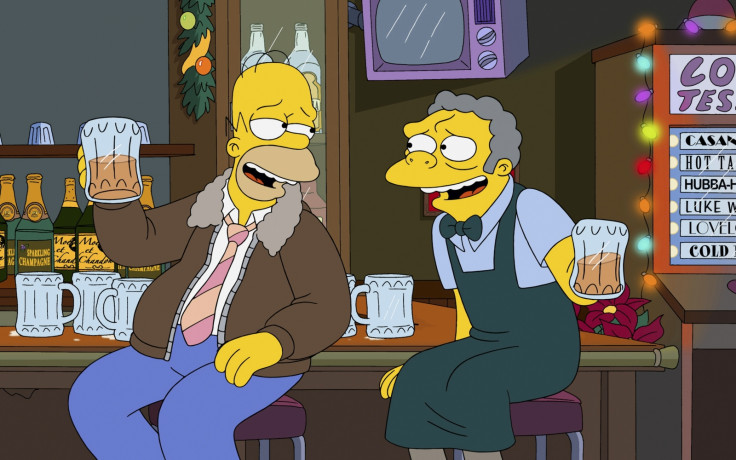
D'oh! You'd never believe it, but in a new research paper, computer scientists at Google DeepMind have admitted that its artificial intelligence technology still struggles to identify many common human behaviours that Homer Simpson exhibits – whether it's eating doughnuts or crisps, falling on his face, yawning or drinking beer.
To try to get the DeepMind AI neural network to understand and recognise human behaviour, researchers created a huge dataset of over 300,000 YouTube video clips showing human actions.
While none of the clips actually featured Homer Simpson, many of the foods, actions and behaviours the system was unable to recognise bore a striking pattern to the animated character's ways, which the researchers had a little fun with.
They also enlisted online workers from Amazon's Mechanical Turk service to look at common types of human behaviour and break it down into a labelled guide containing 400 human action classes, ranging from everything from "brushing hair" to "riding a unicycle" to "playing a violin", with each class corresponding to at least 400 video clips.
When the Kinetics dataset was complete, the researchers then began training the neural network to recognise human actions – a machine learning technique known as "supervised learning".
Neural network struggled with body movements
The problem with artificial intelligence is that, in some cases, computers are still far less smart than even a human baby, so when the researchers played a series of video clips and asked the neural network to identify the human actions displayed, sometimes the neural network became confused.
The neural network found it most difficult to distinguish what exactly humans were eating, whether it was hotdogs, donuts or crisps. It was also unable to understand different dance movements and actions that only affected one part of the body, such as sniffing, sneezing, headbutting, slapping, shaking hands or playing the Rock, Paper, Scissors hand game.
However, the neural network was conversely very good at identifying people presenting the weather forecast; a person riding a mechanical bull; sled dog racing; people playing squash; an individual filling in their eyebrows with makeup; bench presses and pull ups; a baby crawling; someone weaving a basket; horse riding; bowling; or someone swinging from a trapeze.
No inherent gender bias detected
What is a neural network?
Neural networks are large networks of artificially intelligent classical computers that are trained using computer algorithms to solve complex problems in a similar way to the human central nervous system, whereby different layers examine different parts of the problem and combine to produce an answer.
In the last two years, computer scientists have demonstrated that neural networks can process images to produce surprising results, such as psychedelic paintings and amazing selfie filters, or use the images to learn to recognise and detect patterns, such as recognising blurred faces from photographs, or predicting social unrest several days in advance.
However, it is still difficult to teach computers to think like a human, and research continues.
There are increasing concerns that the results produced from big data analytics could have a tendency to be biased and discriminate against a particular subset of people, for example, low income families or certain ethnic groups, due to the data or the language inputs being inherently biased.
The computer scientists analysed the dataset and found that there was no single gender that was more dominant in 340 out of the 400 human action classes, so for example, the neural network was just as able to identify a female playing a game of poker as it would a male.
The open access paper describing how the dataset works, entitled "The Kinetics Human Action Video Dataset" is available on the arXiv preprint server.
"AI systems are now very good at recognising objects in images, but still have trouble making sense of videos. One of the main reasons for this is that the research community has so far lacked a large, high-quality video dataset," a DeepMind spokesperson told IEEE Spectrum.
"Video understanding represents a significant challenge for the research community, and we are in the very early stages with this. Any real-world applications are still a really long way off, but you can see potential in areas such as medicine, for example, aiding the diagnosis of heart problems in echocardiograms."
© Copyright IBTimes 2025. All rights reserved.